Amazon SageMaker AI 客户
了解全球领先的企业如何使用 Amazon SageMaker AI 构建、训练和部署机器学习(ML)模型。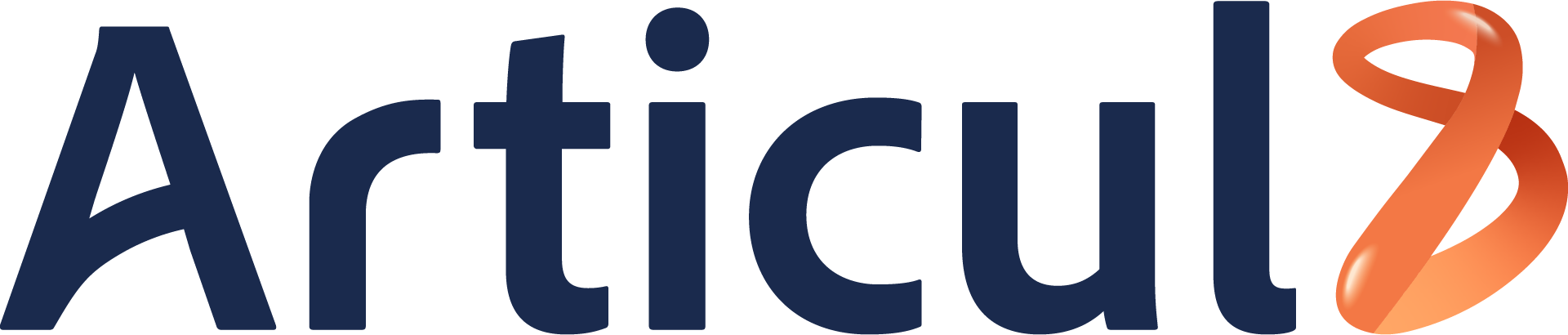
Articul8 AI
“Amazon SageMaker HyperPod 为我们提供了极大的帮助,让我们能够更高效地管理和运营计算资源,同时最大限度地减少停机时间。我们是基于 Slurm 的 HyperPod 服务的早期采用者,并受益于其易用性和弹性特性,使生产力提高了 35%,并快速扩大了我们的 GenAI 运营规模。作为一家以 Kubernetes 为核心的公司,我们很高兴地宣布,Amazon EKS 现已支持 SageMaker HyperPod。这对我们来说是一项颠覆性的技术,因为它与我们现有的训练流程无缝集成,使我们更容易管理和操作我们的大规模 Kubernetes 集群。此外,这也对我们的最终客户有帮助,因为我们现在能够将此功能打包并产品化到我们的 GenAI 平台中,使我们的客户能够以更简化的方式运行自己的训练和微调工作负载。”
Arun Subramaniyan,Articul8 AI 创始人兼首席执行官
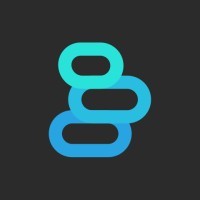
Observea
“作为一家快速发展的初创企业和 AI 研究公司,SageMaker HyperPod 对 Amazon EKS 的支持对于加快我们的产品上市起到了重要作用。借助 SageMaker Hyperpod,我们成功推出了一个稳定且安全的平台,提供容器化的高性能计算(HPC)应用程序,作为面向我们的最终客户的服务,这些最终客户包括顶尖大学的 AI 研究项目、AI 初创企业和传统企业。通过使用 SageMaker HyperPod,我们的客户和内部团队不再需要为 Kubernetes 控制面板的操作和配置而担心,并且 SageMaker HyperPod 提供支持复杂 HPC 工作负载的网络性能和优化配置。借助 SageMaker HyperPod 中的 EKS 支持,我们可以减少在基础设施管理中进行千篇一律的繁重工作所花费的时间,并将运营成本降低 30% 以上。”
Vamsi Pandari,Observea 创始人
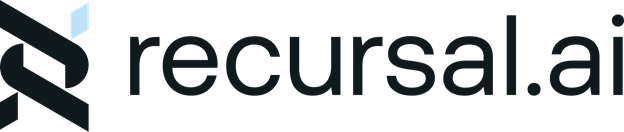
Recursal AI
“整个过程得到了简化。通过使用 SageMaker HyperPod,我们可以利用集群弹性功能,在硬件出现故障时识别问题并自动从上次保存的检查点恢复训练作业。我们以 Kubernetes 为共同主线运行非常多样化的工作负载,包括应用程序、推理和训练。对于我们来说,带有 SageMaker HyperPod 的 Amazon EKS 非常有效:节点会进入我们的集群。”
Nathan Wilce,Recursal 基础设施/数据主管
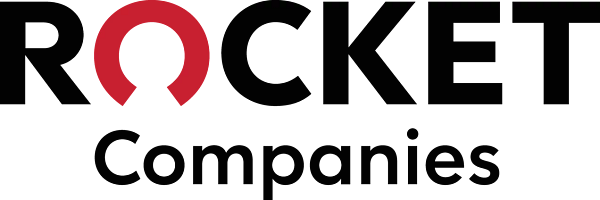
Rocket Mortgage
“Rocket Mortgage 很荣幸能与 AWS 这一重要合作伙伴共同走在将人工智能和数据科学融入购房之旅的前沿。我们正在借助 Amazon SageMaker AI 来改变机器学习运营,提升效率和精确度。SageMaker Pipelines 的视觉编辑器让我们能够通过自动化验证流程快速评测全新开源大型语言模型(LLM)的性能,从而最大限度地减少评估新版本所需的时间,这在快速变化的环境中至关重要。其易用性使我们的数据科学团队能够专注于创新,而非重写代码。”
Shawn Malhotra,Rocket Companies 首席技术官
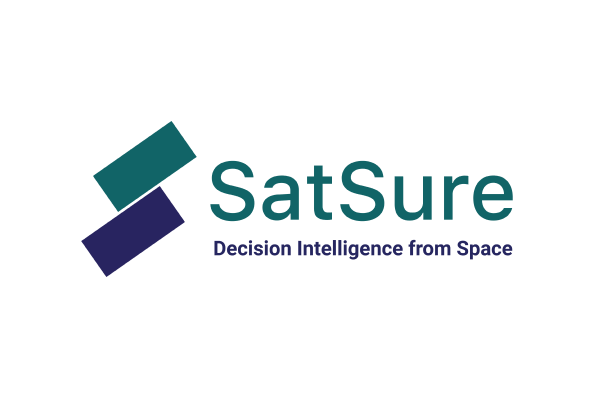
SatSure
SatSure 是地理空间决策智能解决方案领域的全球领导者,它使用地球观测数据和深度学习模型来为各种使用案例提供见解,包括全天候作物监测和农场风险评分、地表覆盖变化检测、植被管理、火灾风险和土地特征识别。
“我们利用 Amazon SageMaker Pipelines 为应用程序创建模型,该模型可在低分辨率卫星图像中识别农业农场边界。从大型卫星图像数据集中开发最先进的深度学习模型极具挑战性。由于 Pipelines 使我们能够自动执行频繁的数据预处理、模型训练和模型部署,因此我们能够更加专注于 AI 创新并减少在手动流程上花费的时间。拖放式用户界面使团队中的新数据科学家无需掌握关于特定工作流程编排框架的专业知识,即可轻松、快速地构建机器学习工作流。”
Prateep Basu,SatSure Ltd. 创始人兼首席执行官
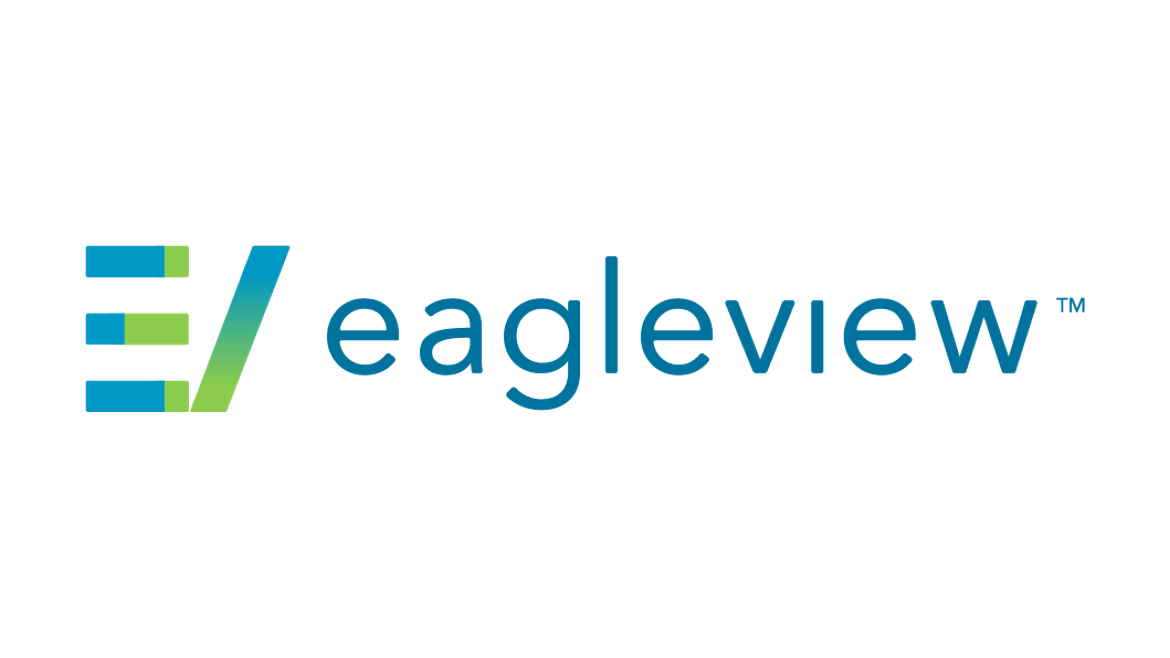
EagleView
“为了满足客户对高质量数据见解的需求,我们在持续研究和提供由机器学习提供支持的新功能。全新的 Amazon SageMaker Pipelines 拖放式用户界面将为我们的数据科学家提供一种方法,使他们能够专注于解决更棘手的计算机视觉(CV)问题,而无需担心 MLOps。我们正在创建 Pipelines,使数据科学家只需在 SageMaker AI 中注册其模型,而无需与 ML 工程师协调以优化模型服务环境。多步骤管道会自动将注册的模型部署到 QA 环境中的 Amazon SageMaker 推理端点进行负载测试,并在获得机器学习工程师的批准后部署到生产环境。我们的端到端机器学习开发的整体速度显著提高,因为 Amazon SageMaker Pipelines 可以轻松与 CI/CD、消息传递服务等其他 AWS 服务集成以构建高度定制的机器学习工作流。”
Garrett Hemann,EagleView 人工智能/机器学习主管
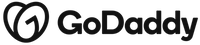
GoDaddy
在 GoDaddy,我们的目标是为普通创业者提供建立业务的工具,帮助他们取得成功。“我们致力于满足客户的不同需求。他们经常通过电子邮件、聊天和社交媒体等多种渠道与他们支持的企业进行全天候沟通。”GoDaddy 应用机器学习和人工智能副总裁 Jing Xi 说道。“如今,生成式人工智能为小型企业营造了一个公平的竞争环境,因为它使中小型企业能够轻松获得通常为大公司预留的极其丰富的功能和知识。但是,我们的生成式人工智能开发团队面临的最大挑战之一是,如何努力找出哪种 FM 适合他们的业务应用程序。对于我们来说,重要的是能够根据对客户最重要的特定标准轻松比较模型,并在模型成本、延迟以及模型准确性和性能之间取得适当的平衡。Amazon SageMaker AI 的新模型评估功能消除了模型选择过程中涉及的复杂性,帮助我们加快从构思到实施的进度,并轻松对这些模型的新版本进行实验、开发、部署和管理。我们很高兴能够在更多团队中扩展对这一新功能的访问,使我们的开发人员能够提高他们的生产力,并进一步释放生成式人工智能的力量,帮助客户发展业务。”
“GoDaddy 在利用机器学习提供以客户为中心的功能以及提高内部运营成本效率方面一直处于领先地位。为了实现这些目标,我们的机器学习科学家实施了多个项目。使用模型注册表和部署快速迭代数据管理、实验记录、模型构件管理的机器学习工作流程对于实现价值至关重要。我们的机器学习科学家明确且强烈地表达了需要一款像 MLflow 这样的定制工具的要求。Amazon SageMaker AI 为机器学习科学家提供了一个受监管的托管平台,用于端到端机器学习工作流程,并通过在 SageMaker AI 中使用 MLflow 等行业标准工具来增强我们的模型开发生命周期。我们获得了 Amazon SageMaker 模型注册表等产品的企业级安全性和成熟度,同时还通过 MLflow 利用行业标准机器学习实验跟踪。在团队内部采用 SageMaker AI 的 MLflow 减少了维护我们自己的 MLflow 托管实例的运营负担,同时保持了我们的交付速度,并且有助于加强协作。我们很高兴从早期阶段就与 SageMaker AI 团队合作,共同完善这一产品,同时为我们的机器学习科学家创造价值。”
Karthik Iyer,工程机器学习总监
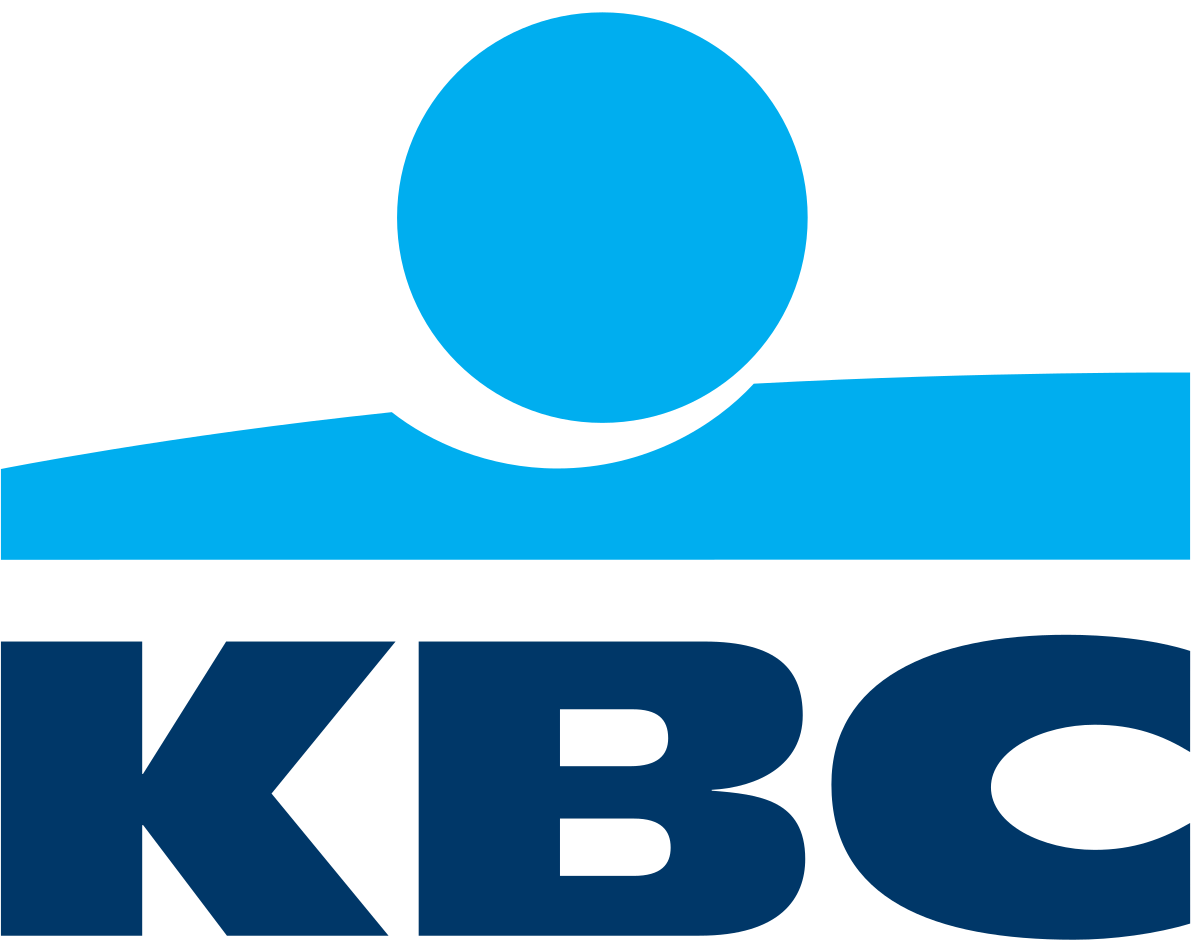
KBC
“在比利时联合银行,我们认为,为我们的数据科学家提供合适的工具对于推动创新至关重要。推动创新的一种有效方法是持续的实验,这使我们能够探索新想法并改进我们的模型。MLFlow 提供了一个强大的平台来管理和记录实验。将其作为托管服务将增强我们数据科学家的用户体验,同时简化我们机器学习平台的设置和维护。”
Thiago Alves,MLOps 工程师
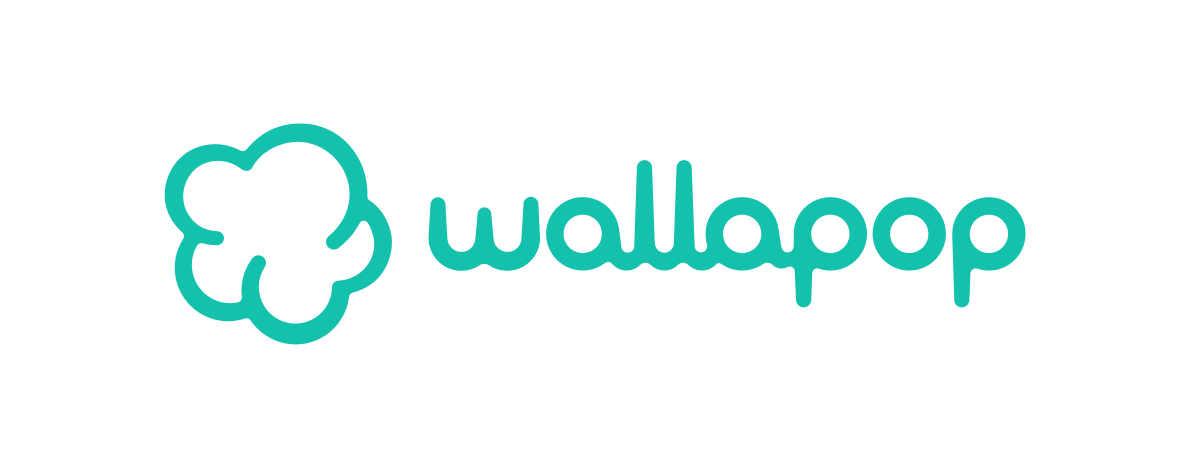
Wallapop
“为了有效管理日益增多的机器学习实验并确保成功部署模型,务必借助一个强大的系统来跟踪实验和注册模型。MLflow 提供了一个可满足此需求的有效解决方案,它能够详细、无缝地跟踪所有机器学习实验,并且只需对我们的代码库进行极少的修改。这有助于为 Wallapop 选择最佳模型,并确保精简、高效的机器学习开发流程。因此,通过在 AWS 内直接集成和管理该平台,我们的团队可以专注于机器学习解决方案的实际价值,而不是执行此类服务所需的各种繁重工作。”
Martí Jordà Roca,机器学习工程师
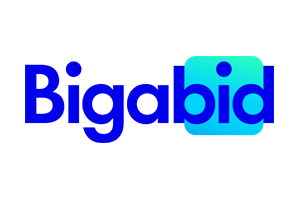
BigaBid
“Amazon SageMaker AI 使我们能够轻松地大规模创建分布式机器学习模型。我们可以在 Amazon SageMaker AI 中无缝自动化大部分开发,而不需要依赖手动流程。我们需要一种可靠的方法来跟踪我们的自动化训练作业的执行情况,以便比较作业、找到最佳模型并将其部署到生产环境中。与 MLflow 的集成使我们能够做到这一点,而无需自行设置和管理 MLflow 所涉及的各种繁重工作。这进一步增强了我们的工作流程,提供了比较模型和模型注册的强大功能,显著提高了我们的开发和部署效率。”
Eyal Trabelsi,数据架构师
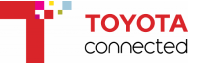
Toyota Connected
“带有 MLflow 的 Amazon SageMaker AI 是与 SageMaker AI 简单而高效的集成,为跟踪和管理实验和模型质量提供了巨大的价值。MLflow 与 Amazon SageMaker AI 的原生集成使我们的模型能够轻而易举地跟踪和推广任务。作为一项托管服务,我们不必为底层基础设施而担心,这使我们可以专注于改进模型和加快开发周期。”
Sumeet Kishnani,管理数据科学家
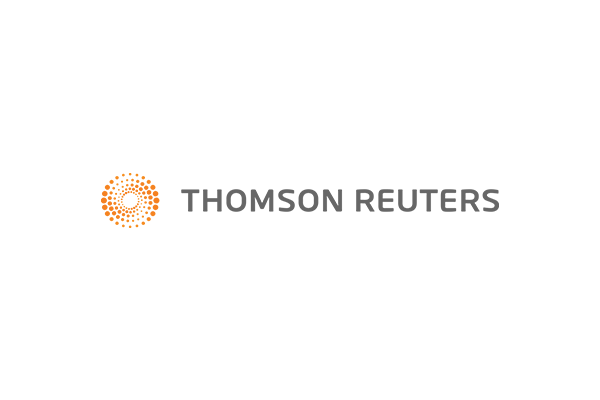
Thomson Reuters
“30 多年来,Thomson Reuters 一直站在 AI 开发的最前沿,我们致力于提供有意义的解决方案,帮助我们的客户更快地交付结果,更好地获取可信信息。为了加速我们在生成式人工智能方面的创新,除了与 LLM 提供商合作外,我们还在探索如何利用我们独特的专有内容和人类专业知识来更有效地训练定制模型。SageMaker HyperPod 的分布式训练库能帮助我们提高大规模模型训练的性能,而且它的弹性功能可以帮助我们节省在基础设施监控和管理上花费的时间。在 SageMaker HyperPod 上训练我们的基础模型将加快我们的上市速度,并帮助我们快速为客户提供优质的解决方案。”
Joel Hron,Thomson Reuters AI 和实验室负责人
“我们能够使用 Amazon SageMaker HyperPod 满足大型语言模型的训练要求。通过在 SageMaker HyperPod 上使用 Amazon EKS,我们能够扩展容量并轻松运行训练作业,从而在法律摘要和分类等领域发挥 LLM 的优势。”
John Duprey,Thomson Reuters 实验室杰出工程师
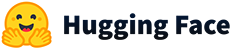
Hugging Face
“Hugging Face 一直在使用 SageMaker HyperPod 创建重要的新型开放基础模型,例如 StarCoder、IDEFICS 和 Zephyr,这些模型的下载量已达数百万次。SageMaker HyperPod 专门构建的弹性和性能功能使我们的开放科学团队能够专注于创新和发布对基础模型构建方式的重要改进,而不是管理基础设施。我们特别喜欢 SageMaker HyperPod 的一点是,它能够检测 ML 硬件故障,并不中断正在进行的模型训练的情况下快速更换有故障的硬件。由于我们的团队需要快速创新,因此自动化作业恢复功能帮助我们最大限度地减少了基础模型训练过程中的中断,让我们在短短一年内节省了数百小时的训练时间。”
Jeff Boudier,Hugging Face 产品主管
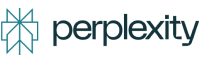
Perplexity AI
“我们一直在寻找合适的机器学习基础设施来提高生产力和降低成本,以便构建高性能的大型语言模型。在进行了几次成功的实验后,我们从其他云提供商转向 AWS,以便使用 Amazon SageMaker HyperPod。在过去的四个月里,我们一直在使用 HyperPod 构建和微调 LLM,为 Perplexity 对话式问答引擎 — 一个可以回答问题并以引文形式提供参考文献的引擎提供支持。由于 SageMaker HyperPod 会自动监控集群运行状况并修复 GPU 故障,因此我们的开发人员能够专注于模型构建,而不需要花时间管理和优化底层基础设施。SageMaker HyperPod 内置的数据和模型并行库帮助我们优化了 GPU 训练时间,并将训练吞吐量提高了一倍。因此,我们的训练实验现在可以以两倍的速度运行,这意味着我们的开发人员可以更快地进行迭代,从而加速为客户开发新的生成式人工智能体验。”
Aravind Srinivas,Perplexity AI 联合创始人兼首席执行官
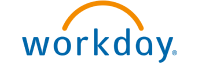
Workday
“全球有超过 1 万个组织依靠 Workday 来管理他们最有价值的资产 — 员工和资金。我们通过选择反映我们公司负责任使用 AI 的政策的最佳基础模型来为客户提供负责任和透明的解决方案。我们针对创建职位描述等必须保持高质量并促进平等机会的任务,测试了 Amazon SageMaker AI 中的新模型评估功能,其跨偏差、质量和性能等指标衡量基础模型的能力让我们感到十分兴奋。期待将来使用这项服务来比较和选择符合我们严格的负责任 AI 标准的模型。”
Shane Luke,Workday AI 和机器学习副总裁
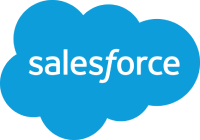
Salesforce
“在 Salesforce,我们采用开放的生态系统方法来构建基础模型,而 Amazon SageMaker AI 是至关重要的组成部分,它可以帮助我们扩展架构并加快上市速度。使用新的 SageMaker AI Inference 功能,我们能够将所有模型部署到单个 SageMaker AI 端点上,该端点可自动处理所有资源分配和计算资源共享,从而提高性能并降低基础模型的部署成本。”
Bhavesh Doshi,Salesforce 工程副总裁。

Bain & Co
“Aura 面临的最大挑战之一是从大量非结构化专业数据中提取有意义的见解。通过使用 Amazon SageMaker Canvas 的大型语言模型,我们实现了数据提取流程自动化,改变了公司评测员工能力和组织结构的方式。这种方法不仅帮助我们扩大了数据分析的规模,还克服了关键字匹配等传统数据分析方法的局限性。利用 SageMaker Canvas 全新的数据准备和 LLM 功能,Aura 能够根据组织结构的有效性、员工技能以及财务业绩对公司进行定量评分和基准测试。”
Purna Doddapaneni,Bain & Co 创始人工作室首席技术官兼合伙人
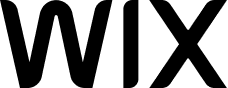
Qred
“通过使用 Amazon SageMaker AI 的集中式平台,保持合规变得更加简单。当我们以集中和安全的方式添加敏感数据时,它会更加简单。”
Lezgin Bakircioglu,Qred 首席技术官
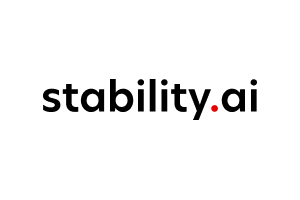
Stability AI
“作为领先的开源生成式人工智能公司,我们的目标是最大限度地提高现代 AI 的可访问性。我们正在构建具有数百亿个参数的基础模型,而这需要能够扩展优化训练性能的基础设施。借助 SageMaker HyperPod 的托管基础设施和优化库,我们可以将训练时间和成本减少 50% 以上。它使我们的模型训练更具弹性和性能,可以更快地构建最先进的模型。”
Emad Mostaque,Stability AI 创始人兼首席执行官
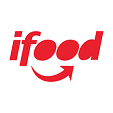
“在 iFood,我们致力于采用机器学习(ML)等技术,通过我们的服务来取悦客户。构建完整、无缝的工作流程来开发、训练和部署模型一直是我们扩展机器学习之旅的关键部分。Amazon SageMaker Pipelines 帮助我们快速构建多个可扩展的自动化机器学习工作流,并让我们轻松有效地部署和管理模型。SageMaker Pipelines 使我们的开发周期更加高效。我们通过 Amazon SageMaker AI 的所有这些新功能继续加强我们在使用 AI/ML 提供卓越客户服务和效率方面的领导地位。”
iFood 首席数据科学家 Sandor Caetano
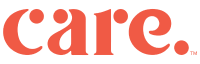
“供需平衡的强大护理行业对于从单个家庭到国家 GDP 的经济增长都至关重要。Amazon SageMaker Pipelines 让我们感到非常兴奋,因为我们相信,它将通过使用一组一致的精选数据,帮助我们在数据科学和开发团队中更好地实现扩展,我们可以使用这些精选数据构建从数据准备到部署的可扩展端到端机器学习 (ML) 模型管道。借助最新公布的 Amazon SageMaker AI 功能,我们可以加快针对不同应用的机器学习模型的开发和部署,通过更快的实时推荐帮助客户作出更明智的决策。”
Clemens Tummeltshammer,Care.com 数据科学部经理
_Logo_Resized.f91898f019610a66c5a9284b90b71cf609deb12e.png)
“通过使用机器学习,3M 正在改进久经考验的产品(如砂纸),并推动其他领域的创新,包括医疗保健。随着我们计划将机器学习扩展到 3M 的更多领域,我们看到数据和模型的数量正在快速增长 – 每年翻一番。我们热衷于新的 SageMaker AI 功能,因为它们将帮助我们实现扩展。Amazon SageMaker Data Wrangler 使准备模型训练数据变得更加容易,并且 Amazon SageMaker Feature Store 消除了重复创建相同模型功能的需要。最后,Amazon SageMaker Pipelines 将帮助我们自动准备数据、构建模型并将模型部署到端到端工作流程中,以便缩短模型的上市时间。我们的研究人员期待着利用 3M 的新科学速度。”
David Frazee,3M 公司系统研究实验室技术主管
“借助 Amazon SageMaker JumpStart,我们能够试验多种基础模型并选择最适合我们医疗保健需求的一个,然后使用 SageMaker 符合 HIPAA 的模型部署快速启动 ML 应用程序。这使我们能够提高处方和客户服务数据输入流程的速度和规模。”
Alexandre Alves,Amazon Pharmacy 高级首席工程师
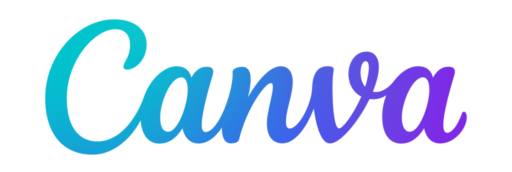
“在 Canva,我们的使命是“赋予世界设计的力量”,让任何人都能轻松地在任何设备上创作精美的内容。借助生成式人工智能,我们正在帮助用户尽可能顺利地将他们的想法变为现实。借助 SageMaker JumpStart,我们使我们的团队能够开始使用生成式人工智能并测试各种基础模型。在我们的全球黑客马拉松中,Canvanauts 能够轻松部署各种基础模型并启动和运行他们的项目。这是我们的黑客马拉松取得成功的重要原因。”
Nic Wittison,Canva 人工智能产品工程主管
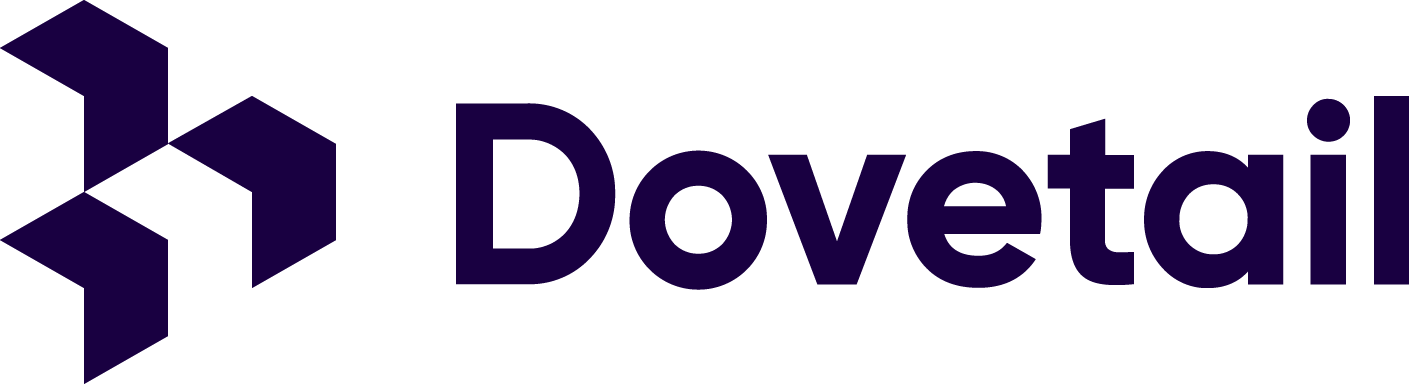
“在 Dovetail,我们通过更好地了解客户需求,帮助组织提高产品和服务的质量。借助 Amazon SageMaker JumpStart,我们可以轻松访问、测试和部署尖端的基础模型。我们使用 AI21 Jurassic-2 Mid 来实现增强的汇总,并能够在几周内将其集成到我们的 SaaS 应用程序中,无需花费数月时间来实施。现在,我们的客户可以高效地从数据中提取并理解洞察,同时维护数据隐私和安全保障。”
Chris Manouvrier,Dovetail 企业架构师经理
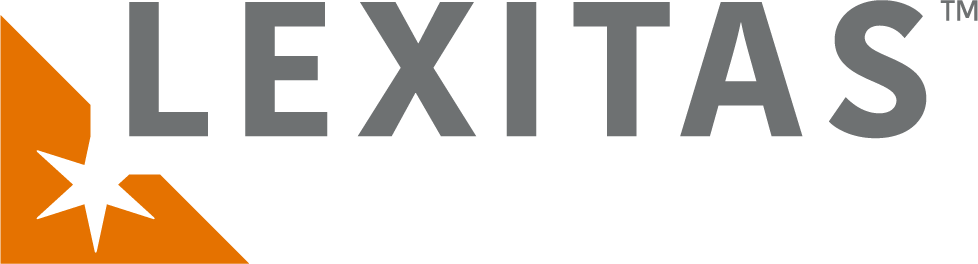
“我们的客户有成千上万份法律文件,解析这些文件的过程既繁琐又耗时。很多时候,没有办法快速获得答案,例如了解谁在证词中提出了问题。现在,借助 Amazon SageMaker JumpStart,我们可以访问最先进的基础模型来为我们的产品提供支持,以便客户可以同时通过数千个文档解处理各种应用场景,例如矛盾检测和语义搜索。律师现在可以利用过去的笔录为未来的事件做好准备,同时保持严格的安全与合规需求。”
Jason Primuth,Lexitas 首席创新官
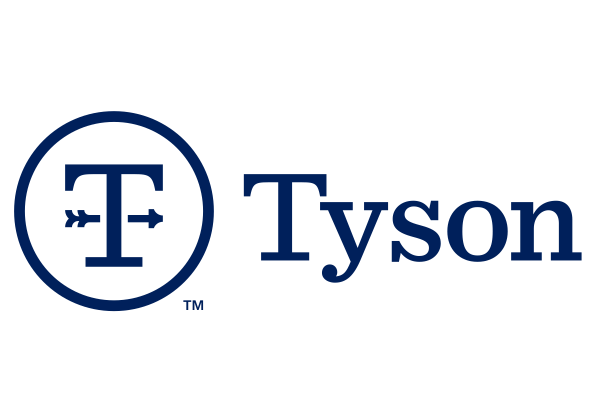
“在 Tyson Foods,我们不断寻找新的方法,以便在生产过程中使用机器学习(ML)来提高生产力。我们使用图像分类模型来识别生产线上需要包装标签的产品。但是,图像分类模型需要使用来自现场的新图像定期进行再训练。Amazon SageMaker JumpStart 使我们的数据科学家能够与支持工程师共享 ML 模型,这样,他们就可以使用新数据训练 ML 模型,而无需编写任何代码。这加快了 ML 解决方案的上市速度,促进了持续改进,并提高了生产力。”
Rahul Damineni,Tyson Foods 专家数据科学家
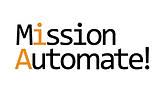
“借助 Amazon SageMaker JumpStart,我们能够在几天之内启动机器学习解决方案,从而更快、更可靠地满足机器学习预测需求。”
Alex Panait,Mission Automate 首席执行官
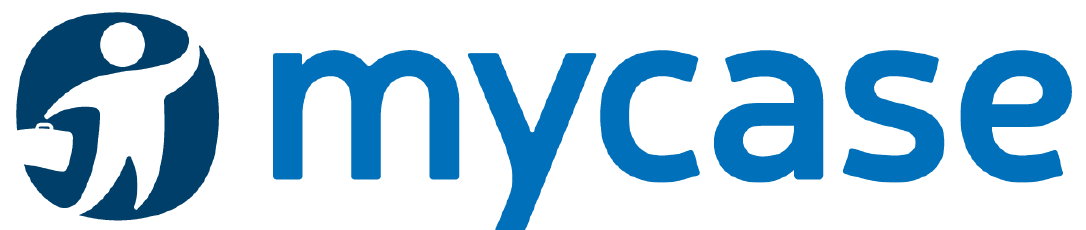
“借助 Amazon SageMaker JumpStart,我们可以获得更好的起点,从而在 4-6 周而不是 3-4 个月内,为我们自己的使用案例部署机器学习解决方案。”
Gus Nguyen,MyCase 软件工程师
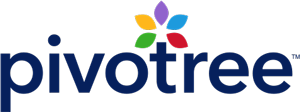
“借助 Amazon SageMaker JumpStart,我们可以更快地构建机器学习应用程序,例如自动异常检测或对象分类,并在几天之内启动从概念验证到生产的解决方案。”
Milos Hanzel,Pivotree 平台架构师
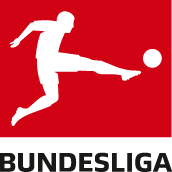
“Amazon SageMaker Clarify 与德甲赛况数字平台的其余部分无缝集成,是在 Amazon SageMaker AI 上标准化 ML 工作流的长期策略的关键部分。通过使用 AWS 的创新技术(例如机器学习)来提供更深入的见解,让球迷更好地理解球场上的瞬间决定,德甲赛况可以让观众更深入地了解每场比赛中的关键决定。”
德国足球职业联盟数字创新执行副总裁 Andreas Heyden
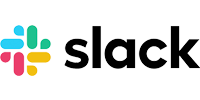
“借助 Amazon SageMaker JumpStart,Slack 可以访问最先进的基础模型,在注重安全和隐私的同时为 Slack AI 提供支持。Slack 客户现在可以更智能地进行搜索、即时总结对话并实现最高生产力。”
Slack 人工智能产品副总裁 Jackie Rocca
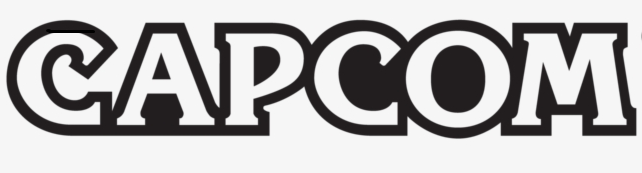
“AutoGluon 和 Amazon SageMaker Clarify 的结合使我们的客户流失模型能够以 94% 的准确率预测客户流失。SageMaker Clarify 通过 SHAP 值提供可解释性来帮助我们了解模型行为。通过 SageMaker Clarify,与本地计算相比,我们将 SHAP 值的计算成本降低了高达 50%。联合解决方案使我们能够更好地了解模型并以更高的准确率提高客户满意度,同时节省大量成本。”
CAPCOM 数据组主管 Masahiro Takamoto
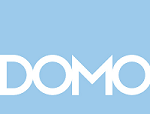
“Domo 提供了一套可扩展的数据科学解决方案,企业中的任何人都可以轻松使用和了解。借助 Clarify,我们的客户可以了解他们的 AI 模型如何进行预测。Clarify 与 Domo 的结合有助于为我们的客户提高 AI 速度和智能,为业务和生态系统中的每个人提供 AI 的强大功能。”
Domo AI 和数据科学主管 Ben Ainscough 博士
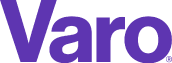
Varo Bank 是总部位于美国的数字化银行,该银行使用 AI/ML 帮助进行快速基于风险的决策,以向客户提供其创新产品和服务。
“Varo 对 ML 模型的可解释性和透明度投入很大,我们很高兴地看到 Amazon SageMaker Clarify 的结果在推动这些努力。”
Varo Money 数据科学主管 Sachin Shetty

LG AI Research 旨在通过使用 Amazon SageMaker AI 更快地训练和部署 ML 模型,引领下一个 AI 时代。
“我们最近推出了 Tilda,一个由 EXAONE 提供支持的 AI 艺术家,EXAONE 是一个超级巨型人工智能系统,可以处理 2.5 亿高清图文对数据集。多模态人工智能使 Tilda 能够自行创建新图像,并且能够超越其感知的语言进行探索。Amazon SageMaker AI 对于 EXAONE 的开发至关重要,因为它具有可扩展性和分布式训练功能。具体来说,由于训练这个超巨型人工智能需要大量计算,因此高效的并行处理非常重要。我们还需要持续管理大规模数据并灵活响应新获取的数据。通过使用 Amazon SageMaker AI 模型训练和分布式训练库,我们优化了分布式训练,并将模型训练速度提高了 59%,而且无需对训练代码进行重大修改。”
Seung Hwan Kim,LG AI Research 副总裁兼视觉实验室负责人
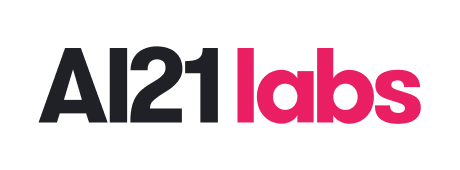
“在 AI21 Labs,我们帮助企业和开发人员使用尖端的语言模型来重塑用户与文本的交互方式,并且不需要他们具备 NLP 专业知识。 我们的开发者平台 AI21 Studio 提供对文本生成、智能摘要甚至代码生成的访问,所有这些都基于我们的大型语言模型系列。我们最近训练的具有 170 亿个参数的 Jurassic-Grande(TM)模型是使用 Amazon SageMaker AI 训练的。Amazon SageMaker AI 使模型训练过程更加简单、高效,并且与 DeepSpeed 库完美配合。因此,我们能够轻松地将分布式训练作业扩展到数百个 Nvidia A100 GPU。Grande 模型提供的文本生成质量与我们更大的 1780 亿参数模型相当,而推理成本却低得多。因此,我们在生产环境中部署 Jurassic-Grande 的客户能够每天为数百万实时用户提供服务,并在不牺牲用户体验的情况下享受单元经济效益改善的优势。”
Dan Padnos,AI21 Labs 架构副总裁
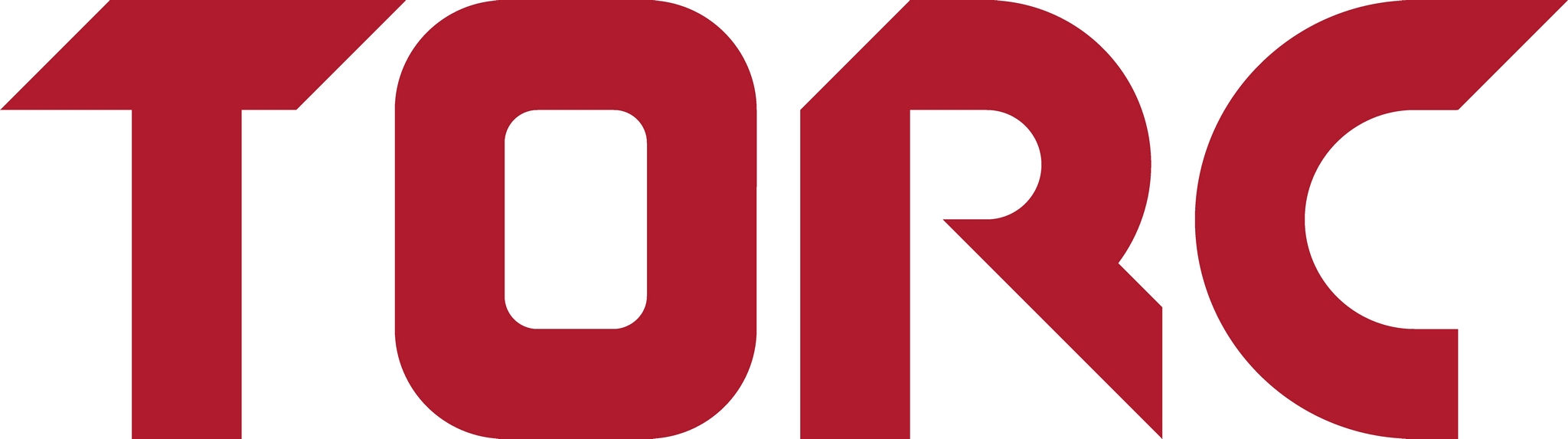
作为自 2005 年以来,一直在自动驾驶汽车领域处于领先地位的企业,Torc.ai 借助 Amazon SageMaker AI 和 Amazon SageMaker AI 分布式数据并行(SMDDP)库,致力于将自动驾驶卡车商业化,为货运行业提供更安全、更持久的长途运输解决方案。
“我的团队现在能够使用 Amazon SageMaker AI 模型训练和 Amazon SageMaker AI 分布式数据并行(SMDDP)库轻松运行大规模分布式训练作业,其中涉及 TB 级的训练数据和具有数百万个参数的模型。Amazon SageMaker AI 分布式模型训练和 SMDDP 可帮助我们无缝扩展,而无需管理训练基础设施。它将我们训练模型的时间从几天缩短到几个小时,使我们能够压缩设计周期,并且以比以往任何时候都更快的速度为我们的车队带来新的自动驾驶汽车功能。”
Derek Johnson,Torc.ai 工程副总裁

下一代网络安全解决方案和服务的全球领导者 Sophos 使用 Amazon SageMaker AI 更有效地训练其 ML 模型。
“我们强大的技术可以检测并消除狡猾地掺杂恶意软件的文件。然而,使用 XGBoost 模型来处理数 TB 大小的数据集非常耗时,有时由于内存空间有限,根本无法进行处理。借助 Amazon SageMaker AI 分布式训练,我们可以成功训练轻量级 XGBoost 模型,该模型占用的磁盘空间(最多小 25 倍)和内存空间(最多小 5 倍)比其前代模型小得多。使用 Amazon SageMaker AI 自动模型调优和竞价型实例的分布式训练,我们可以快速、更有效地修改和重新训练模型,而无需调整扩展到如此大的数据集所需的底层训练基础设施。”
Konstantin Berlin,Sophos 人工智能主管
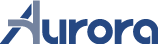
“Aurora 先进的机器学习和大规模模拟是我们安全快速地进行技术开发的基础,AWS 则提供了我们持续推进所需的高性能。凭借几乎无限的扩展能力,AWS 支持数百万次虚拟测试,以验证 Aurora 的功能,使其能够安全地应对现实世界驾驶的无数边缘情况。”
Chris Urmson,Aurora 首席执行官

“我们使用计算机视觉模型进行场景分割,这对于场景理解很重要。过去,训练一个训练周期的模型需要 57 分钟,这拖慢了我们的速度。使用 Amazon SageMaker AI 的数据并行库并借助 Amazon ML Solutions Lab,我们只需 6 分钟即可在 5ml.p3.16xlarge 实例上使用优化的训练代码进行训练。由于训练时间缩短了 10 倍,因此我们可以在开发周期中花更多时间准备数据。”
Jinwook Choi,Hyundai Motor Company 高级研究工程师
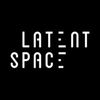
“在 Latent Space,我们正在构建一个神经渲染游戏引擎,任何人都可以快速地随心创建。在语言建模方面的进步的推动下,我们正在努力结合对文本和图像的语义理解,确定要生成的内容。我们目前的重点是利用信息检索来增强大规模的模型训练,我们对此拥有复杂的机器学习管道。由于要同时训练多个数据源和模型,所以该设置为分布式训练带来了挑战。因此,我们正在利用 Amazon SageMaker AI 中的新分布式训练功能,高效地扩展大型生成模型的训练。”
Sarah Jane Hong,Latent Space 联合创始人兼首席科学官
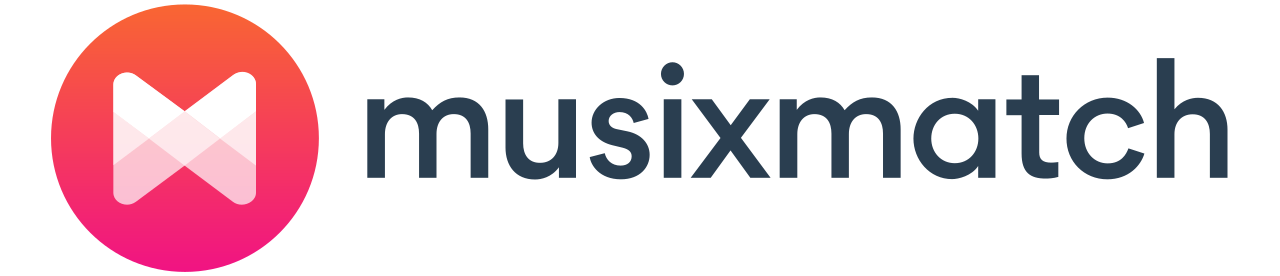
“Musixmatch 使用 Amazon SageMaker AI 构建自然语言处理(NLP)和音频处理模型,并且正在通过 Amazon SageMaker AI 试用 Hugging Face。我们之所以选择 Amazon SageMaker AI,是因为它支持数据科学家以迭代方式快速构建、训练和调整模型,而不必担心管理底层基础设施,这意味着数据科学家可以更快、更独立地工作。随着公司的发展,我们对训练和调整更大、更复杂的 NLP 模型的要求也越来越高。我们一直在寻找加快训练速度,同时降低训练成本的方法,这就是我们对 Amazon SageMaker Training Compiler 感到兴奋的原因。SageMaker Training Compiler 提供了在训练过程中更有效地使用 GPU 的方法,并且通过 SageMaker Training Compiler、PyTorch 和 Hugging Face 等高级库之间的无缝集成,我们发现基于 Transformer 的训练时间有了显著改善。模型的运行时间从几周缩短到几天,并且训练成本更低。”
Loreto Parisi,Musixmatch 人工智能工程总监
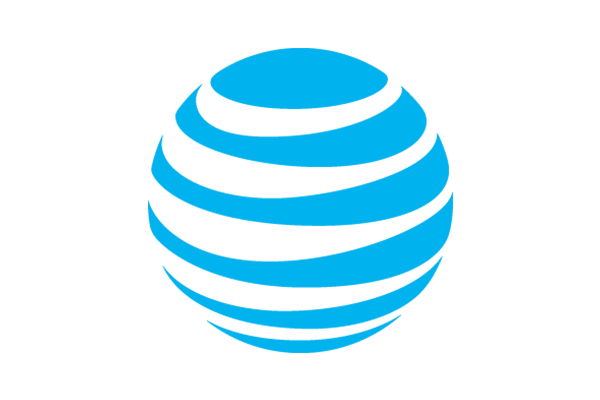
AT&T Cybersecurity 改进了需要使用 Amazon SageMaker AI 多模型终端节点进行近实时预测的威胁检测。
“Amazon SageMaker AI 多模型终端节点不仅经济高效,而且还通过简化模型存储方式为我们带来了一些性能提升。”
Matthew Schneid,AT&T 首席架构师
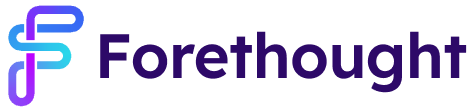
Forethought Technologies 是一家为客服人员提供生成式人工智能解决方案的提供商,公司通过使用 Amazon SageMaker AI,将成本降低了高达 80%。
通过迁移到 Amazon SageMaker AI 多模型终端节点,我们将成本降低了高达 66%,同时为客户提供更短的延迟和更快的响应速度。”
Jad Chamoun,Forethought Technologies 核心工程总监
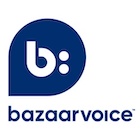
Bazaarvoice 使用 SageMaker 无服务器推理功能将 ML 推断成本降低了 82%
“通过使用 SageMaker Serverless Inference,我们可以大规模高效地进行机器学习,以合理的成本和较低的运营开销快速推出大量模型。”
Lou Kratz,Bazaarvoice 首席研究工程师
Amazon Pharmacy
“借助 Amazon SageMaker JumpStart,我们能够试验多种基础模型并选择最适合我们医疗保健需求的一个,然后使用 SageMaker 符合 HIPAA 的模型部署快速启动 ML 应用程序。这使我们能够提高处方和客户服务数据输入流程的速度和规模。”
Alexandre Alves,Amazon Pharmacy 高级首席工程师
Intuit
“借助 Amazon SageMaker AI,我们可以通过在该平台上构建和部署我们自己的算法来大规模加快人工智能计划的实施。我们将创建新的大规模机器学习和 AI 算法,并将它们部署到该平台上,来解决推动客户发展需要解决的复杂问题。”
Intuit 首席数据官 Ashok Srivastava
GE Healthcare
利用硬件、软件和生物技术的数据和分析,GE Healthcare 正在通过为提供商和患者提供更好的结果来推动医疗保健行业的变革。
“借助 Amazon SageMaker AI,GE Healthcare 可以通过强大的人工智能工具和服务来促进患者护理的改善。Amazon SageMaker AI 的可扩展性以及它与 AWS 服务的原生集成,为我们增加了巨大的价值。我们期待看到 GE Health Cloud 和 Amazon SageMaker AI 之间的持续协作将如何为我们的医疗保健提供商合作伙伴带来更好的结果,并提供更好的患者护理。”
GE Healthcare AI 工程负责人 Sharath Pasupunuti
ADP, Inc.
ADP 是一家领先的全球技术公司,提供人力资本管理 (HCM) 解决方案。ADP DataCloud 利用 ADP 收集的来自 3000 多万员工的优质人力数据,来提供可帮助高管制定实时决策的可行见解,以便更好地管理其业务。
“留住和吸引人才并非易事,因此我们利用人工智能功能不断增强 ADP DataCloud,以帮助雇主维持强大的团队。我们使用 AWS 机器学习(包括 Amazon SageMaker AI)来快速识别人力模式,并在结果(例如员工流动或加薪影响)发生之前进行预测。通过将 AWS 用作人工智能和机器学习的主要平台,我们将部署机器学习模型的时间从 2 周减少到了 1 天。”
Jack Berkowitz,ADP, Inc. 产品开发副总裁
BASF Digital Farming
BASF Digital Farming 有使命帮助农民制定更明智的决策,为克服养活不断增加的全球人口的挑战做出贡献,同时还要减少环境足迹。
“Amazon SageMaker AI 和相关的 AWS 技术支持快速试验,并提供简单易用的功能和 API 以降低 ML 采用的门槛。如此一来,我们可以快速发掘 ML 使用案例的完整价值潜力。”
Christian Kerkhoff博士,数据自动化经理 – BASF Digital Farming GmbH
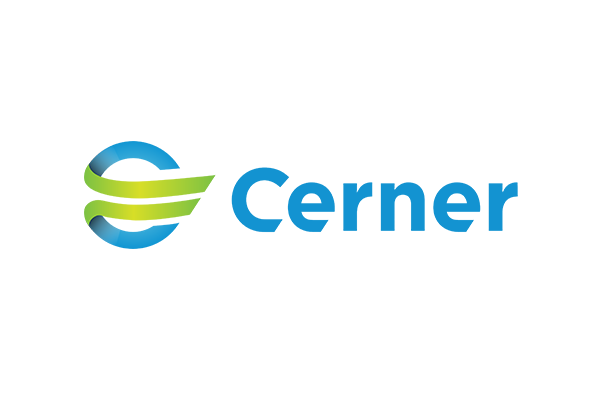
Cerner
Cerner Corporation 是一家全球性的健康和技术公司,提供各种健康信息技术 (HIT) 解决方案、服务、设备和硬件。
“Cerner 为在广泛的临床、财务和运营领域中推动人工智能和机器学习创新而感到自豪。凭借 Cerner 的机器学习生态系统和 Cerner 的自然语言处理所创建的新功能,以及我们与 AWS 合作实现的新功能,我们正在为所有客户加速可扩展创新。Amazon SageMaker AI 是 Cerner 能够通过 AI/ML 按我们的意图向客户提供价值的重要因素之一。此外,Amazon SageMaker AI 为 Cerner 提供了利用 TensorFlow 和 PyTorch 等不同框架,以及与各种 AWS 服务集成的能力。”
Sasanka Are,博士,副总裁 – Cerner
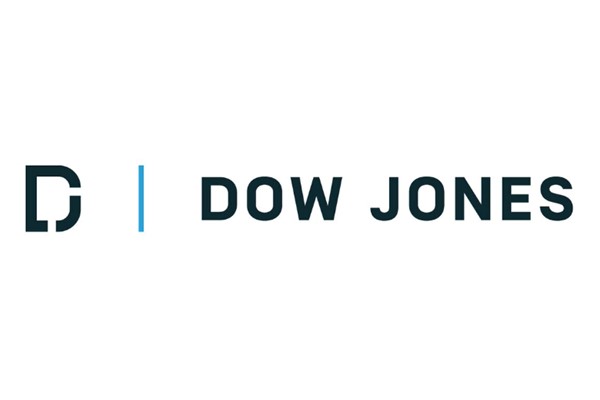
Dow Jones
Dow Jones & Co. 是全球新闻和商业信息提供商,通过报纸、网站、移动应用程序、视频、新闻通讯、杂志、专有数据库、会议和无线电广播为消费者和企业提供内容。
“随着 Dow Jones 继续专注于将机器学习与我们的产品和服务集成,我们发现 AWS 一直是出色的合作伙伴。在我们近期举办的“机器学习黑客马拉松”活动的准备过程中,AWS 团队为参与者提供了有关 Amazon SageMaker AI 和 Amazon Rekognition 的培训,并为所有团队提供全天候支持。结果是,我们的团队就如何应用机器学习提出了一些不错的想法,并且我们将根据其中多个想法在 AWS 上继续开发。该活动取得了巨大成功,同时也是良好合作伙伴关系的一个例子。”
Dow Jones 首席产品和技术官 Ramin Beheshti
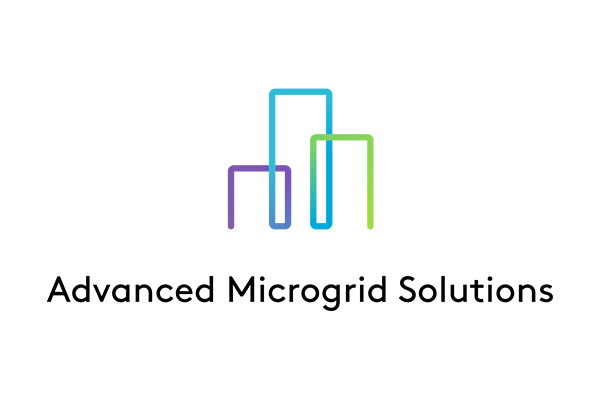
Advanced Microgrid Solutions
Advanced Microgrid Solutions (AMS) 是一家能源平台和服务公司,旨在通过促进清洁能源资产的部署和优化,加速全球向清洁能源经济的转型。NEM 使用现货市场,各方可以在其中进行竞价,以便实现每 5 分钟消耗/供应能源。这需要预计需求预测并在几分钟内进行动态竞价,同时还要处理大量的市场数据。为了解决这一挑战,AMS 使用 TensorFlow 在 Amazon SageMaker AI 上构建了深度学习模型。他们利用 Amazon SageMaker AI 的自动模型调优功能来发现最佳模型参数,并在短短几周内建立模型。他们的模型使所有能源产品在净能源计量方面的市场预测都有所改善,这将转化为显著的效率提升。
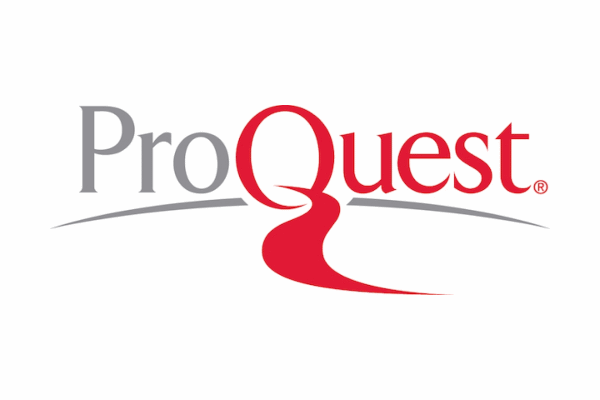
ProQuest
ProQuest 策划全球最大的期刊、电子书、原始材料、论文、新闻和视频,并构建强大的工作流程解决方案,帮助图书馆获取和增长其收藏。ProQuest 产品和服务应用于 150 个国家/地区的高等院校图书馆、幼儿园、小学和中学图书馆、公共图书馆以及企业和政府图书馆。
“我们正在与 AWS 合作,为图书馆读者打造更具吸引力的视频用户体验,使他们在搜索时能返回相关度更高的结果。通过与 AWS ML Solutions Lab 合作,我们使用 Amazon SageMaker AI 测试了不同算法、通过超参数优化调整了模型并对机器学习(ML)模型的部署进行了自动化。我们对目前的结果感到满意,现在正在考虑将 ML 技术应用于其他产品。”
ProQuest 研究工具、服务和平台副总裁 Allan Lu
Celgene
Celgene 是一家全球生物制药公司,致力于改善全球患者的生活。该公司重点关注癌症、免疫炎症和其他医学未攻克疾病的患者,为他们发现、开发创新疗法并实现商业化。
“Celgene 的愿景是提供真正创新和改变生活的治疗方法,改善全球患者的生活质量。有了 Amazon SageMaker AI 和 Apache MXNet,与以往相比,我们能够更快、更轻松地构建和训练深度学习模型来开发解决方案和流程,并且可以轻松扩展我们的工作,发现治疗方法并生产药物。利用 SageMaker AI 和 Amazon EC2 P3 实例,我们加快提供时间训练模型并提高了生产率,让我们的团队能够专注于突破性的研究和发现。”
Celgene 董事 Lance Smith
Atlas Van Lines
Atlas Van Lines 是由运输和储存行业的一群企业家于 1948 年创立,是北美第二大长途货运公司。该组织的发展目标是,在遵循商业黄金法则的同时从一个海岸扩展到另一个海岸。除了广泛的业务范围外,Atlas 还具有超越该行业要求的严格的代理质量要求。
在繁忙的货运季节,Atlas 代理网络在各个市场相互协作,以满足客户需求。过去,他们需要大量人力和劳力来预测容量。他们凭借的是智慧和对资源的直觉以及多年的经验。Atlas 拥有 2011 年以来的历史数据,希望找到一种能够根据未来的市场需求动态调整容量和价格的方法。
Atlas 与 APN 核心级咨询合作伙伴 Pariveda Solutions 合作,使长途货运行业的主动容量和价格管理成为可能。Pariveda 准备了数据,开发并评估了机器学习模型,并调整了性能。他们使用 Amazon SageMaker AI 训练和优化模型,然后使用 Amazon SageMaker AI 的模块化特性将其导出,以使用 Amazon EC2 运行。
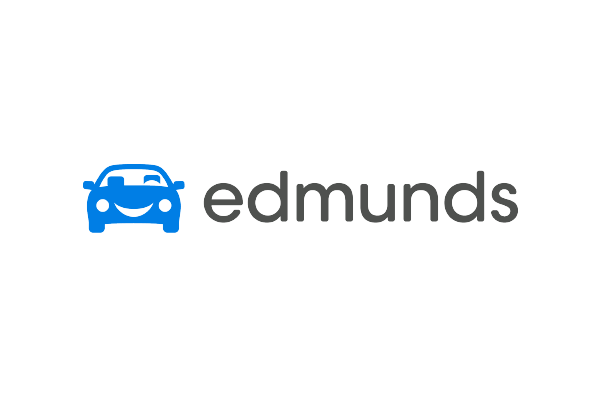
Edmunds
Edmunds.com 是一个汽车销售网站,提供有关车辆的详细信息,并且会不断更新,每月的访客达到 2000 万。
“我们有一项战略举措,那就是让我们的所有工程师都能利用机器学习技术。Amazon SageMaker AI 是帮助我们实现这一目标的关键,它使工程师能够更轻松地大规模构建、训练和部署机器学习模型和算法。我们期待看到 Edmunds 将如何在组织内使用 SageMaker AI 为我们的客户构建新的创新型解决方案。”
Edmunds.com 首席信息官 Stephen Felisan
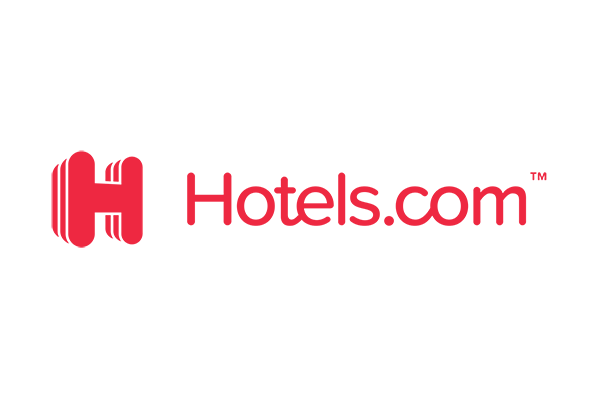
Hotels.com
Hotels.com 是一个领先的全球酒店品牌,运营着 90 个支持 41 种语言的本地化网站。
“Hotels.com 愿意尝试各种方法来推动发展,以利用最新技术并保持创新。借助 Amazon SageMaker AI 的分布式训练、优化算法和内置的超级参数功能,我的团队可以基于我们最大的数据集快速构建更准确的模型,为将模型投产节约了大量时间。只需要一个简单的 API 调用,Amazon SageMaker AI 将显著降低机器学习的复杂性,使我们能够快速创造更好的客户体验。”
Hotels.com 和 Expedia Affiliate Network 副总裁兼首席数据科学官 Matt Fryer
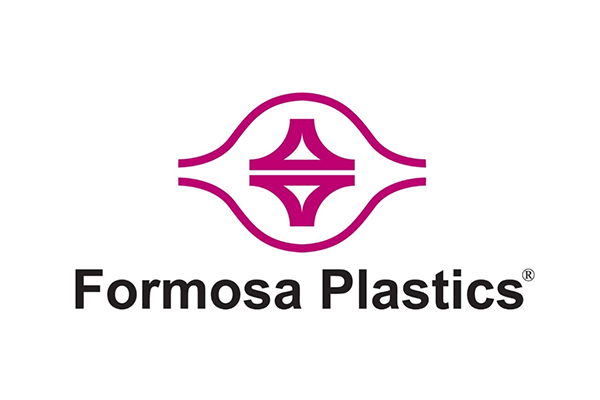
台塑
台湾塑胶工业股份有限公司是一家不断发展的垂直整合塑料树脂和石化产品供应商。台塑提供全系列的聚氯乙烯、聚乙烯和聚丙烯树脂、苛性钠和其他石化产品,可提供客户所需的一致性、性能和质量。
“台塑是台湾顶级石化公司之一,是世界领先的塑胶制品制造商之一。我们决定探索机器学习,以便更准确地检测缺陷并降低人工劳动成本,我们选择 AWS 作为首选云提供商来帮助我们实现这一目标。AWS ML Solutions Lab 与我们一起完成了流程的每个步骤,从发现研讨会到定义业务使用场景,到构建和选择适当的 ML 模型,再到实际部署。使用 Amazon SageMaker AI,机器学习解决方案减少了一半的员工人工检查时间。借助 Solutions Lab 的帮助,我们现在能够在条件变化时自行优化 SageMaker AI 模型。”
台湾塑胶工业股份有限公司副总裁助理 Bill Lee
Voodoo
Voodoo 是一家领先的移动游戏公司,拥有 20 多亿的游戏下载量和 4 亿多每月活跃用户 (MAU)。该公司运营自己的广告平台,并使用机器学习来提高展示给用户的广告出价的准确性和质量。
“在 Voodoo,我们需要确保活跃玩家群体人数过百万且不断扩增。通过在 AWS 上实现机器学习和人工智能工作负载标准化,我们能够按照所需的速度和规模进行迭代,以不断发展业务并吸引玩家参与。利用 Amazon SageMaker AI,我们可以实时决定应向玩家展示哪种广告,并且我们的 3000 多万用户每天会调用端点超过 1 亿次,相当于每天近十亿次预测。借助 AWS 机器学习,我们能够在不到一周的时间内,在小型团队的支持下将准确的模型投入生产,并且能够在此基础上随着团队和业务增长不断进行构建。”
Aymeric Roffé,Voodoo 首席技术官
Regit
Regit 以前称为 Motoring.co.uk,是一家汽车技术公司,同时也是英国的一项领先的针对驾驶者的在线服务。他们提供基于汽车牌照的数字汽车管理服务,并向司机提供信息提醒,如交通运输部 (MOT) 税务、保险和召回。
Regit 与 APN 高级咨询合作伙伴 Peak Business Insight 合作,应用可同时处理类别和可变数据的“类别机器学习模型”,以预测用户更换汽车的可能性,从而为 Regit 带来销量。
Peak 使用 AWS 产品(例如 Amazon SageMaker AI)进行实时摄取、建模和数据输出。Amazon SageMaker AI 每天为 Regit 处理 5000 个 API 请求,根据相关数据要求无缝扩展和调整,并管理销售线索评分结果的交付。同时,Amazon Redshift 和 Amazon Elastic Compute Cloud(Amazon EC2)实例会高效并持续优化模型性能和结果。在 Peak 的帮助下,Regit 已经能够预测其 250 万用户中的哪一个将在何时买车。这意味着他们能以更加个性化和更具针对性的方式为客户提供服务,从而使呼叫中心的收入增加四分之一以上。
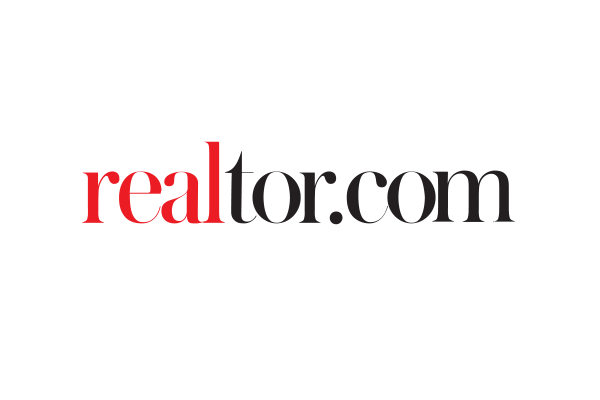
Realtor.com
Move, Inc. 网络(包括 realtor.com®、Doorsteps® 和 Moving.com™)可以在一系列网站和移动设备上为消费者和房地产专业人士提供房地产信息、工具和专业知识。
“我们认为,在我们帮助消费者拥有自己的住房的过程中,Amazon SageMaker AI 是对 realtor.com® 工具集的变革性补充。过去需要很长时间的机器学习工作流程(如训练和优化模型)的效率提高了,并且可由更多开发人员来完成,这使我们的数据科学家和分析师能够专注于为用户创造最丰富的体验。”
Move, Inc 首席数据官兼高级副总裁 Vineet Singh
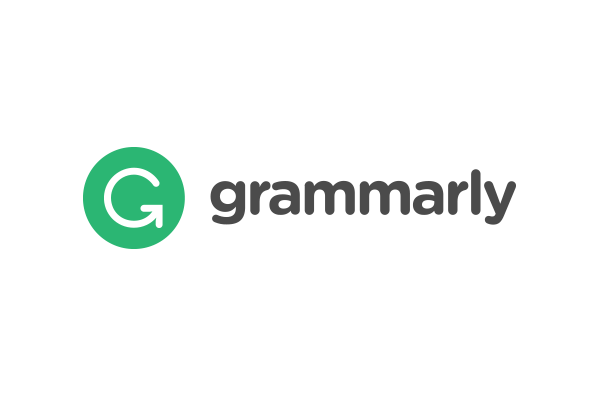
Grammarly
通过结合运用自然语言处理和先进的机器学习技术,Grammarly 的算法在跨设备的多个平台上提供书写帮助,每天帮助数百万人更有效地通信。
“Amazon SageMaker AI 使我们能够在分布式训练环境中开发 TensorFlow 模型。我们的工作流程还与 Amazon EMR 集成以进行预处理,因此我们可以从 Amazon S3 获取数据,并通过 Jupyter Notebook 使用 EMR 和 Spark 过滤数据,然后使用同一笔记本在 Amazon SageMaker AI 中进行训练。SageMaker AI 还具有灵活性,能够满足我们不同的生产要求。我们可以在 SageMaker AI 上运行推理;如果我们只需要模型,则可以将其从 S3 中下载下来,并运行针对 iOS 和 Android 客户的移动设备实施的推理。”
Grammarly 技术主管 Stanislav Levental
Slice Labs
Slice Labs 总部位于美国纽约,业务遍及全球,是第一家按需保险云平台提供商。Slice 通过个人按需保险产品来服务 B2C 市场,通过支持企业建立直观的数字化保险产品来服务 B2B 市场。
“在 Slice,我们深知客户保险需求的持续变化性质,我们之所以选择 AWS 作为我们的首选云平台,是因为它的服务种类极其丰富、灵活性佳,在保险公司中的声誉良好。我们使用了多种 AWS 服务来支持我们的业务,包括借助 AWS 机器学习根据客户的需求将客户与最佳的保险选择对接。在与希望建立并推出智能保险产品的保险公司和高科技公司合作过程中,我们看到了使用 AWS 的巨大成本节省和效率提升优势。例如,我们的采购周期从 47 天缩短到 1 天,节省时间 98%。我们很高兴能不断扩大与 AWS 在区域以及云使用量方面的合作。”
Philippe Lafreniere,Slice Labs 首席增长官
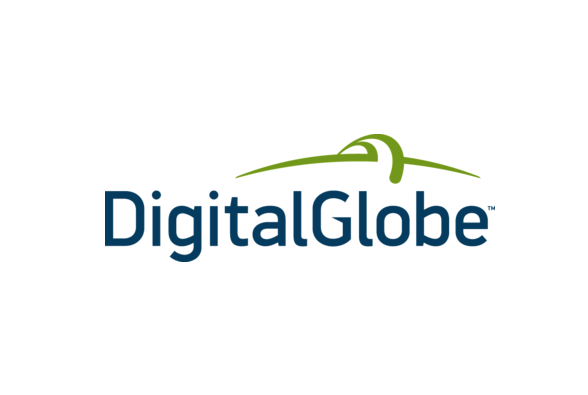
DigitalGlobe
作为高分辨率地球图像、数据和分析的世界领先提供商,DigitalGlobe 每天都需要处理海量数据。
“作为高分辨率地球图像、数据和分析的世界领先提供商,DigitalGlobe 每天都需要处理海量数据。借助 DigitalGlobe,人们可以更轻松地查找、访问我们总容量达 100PB 的图像库(存储在 AWS 云中),并对其运行计算,从而将深度学习应用于卫星图像。我们计划使用 Amazon SageMaker AI,借助托管 Jupyter 笔记本针对 PB 级的地球观测图像数据集训练模型,以使 DigitalGlobe 的 Geospatial 大数据平台(GBDX)用户只需按下一个按钮,创建一个模型,即可将其大规模部署到一个可扩展的分布式环境中。”
Walter Scott博士,Maxar Technologies 首席技术官兼 DigitalGlobe 创始人
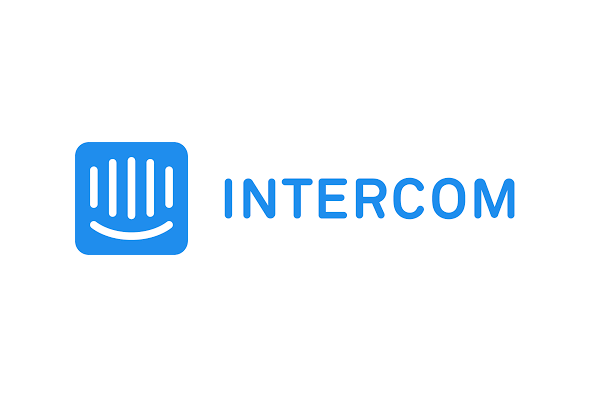
Intercom
Intercom 的消息传送优先产品与其他公司的网站和移动应用程序无缝集成,帮助他们获取、接触客户并为其提供支持。公司成立于 2011 年,在旧金山、伦敦、芝加哥和都柏林设有办事处。
“在 Intercom,我们拥有一支不断成长的数据科学家和以数据为导向的工程师团队,我们经常需要快速迭代,探索新的数据驱动型产品解决方案。在使用 Amazon SageMaker AI 以前,我们尝试了多种不同的方案来构建这些产品,但每种方案都有这样那样的挑战 – 难以实现代码共享,大数据集测试速度非常缓慢,在我们自己的环境中预置和管理硬件也有诸多问题。使用 SageMaker AI 后,所有这些问题都迎刃而解。特别是,我们使用它来为我们的搜索平台和机器学习功能开发算法,发现 SageMaker AI 的托管 Jupyter 笔记本可帮助我们快速构建和迭代。特别关键的是,SageMaker AI 这种托管式的服务可让我的团队专注于手头的任务,无需他顾。对 Intercom 而言,Amazon SageMaker AI 是一个极有价值的服务,我们很高兴随着我们公司的成长越来越多地使用它。”
Intercom 机器学习部门高级数据科学家 Kevin McNally
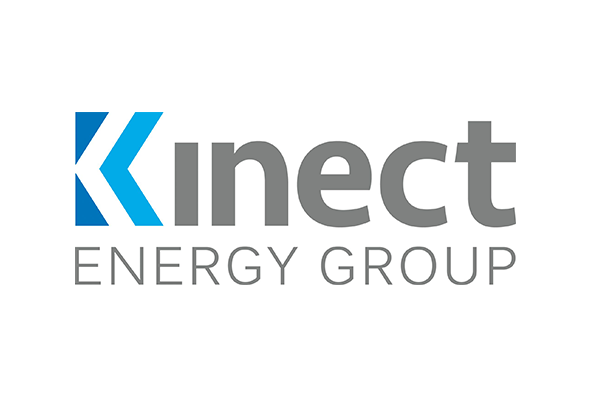
Kinect Energy Group
Kinect Energy Group 是 World Fuel Services 的一家子公司,World Fuel Services 是一家财富 100 强企业,主要通过空运、海运和陆运方式,为商业和工业客户提供能源采购咨询服务、供应履行、交易和支付管理解决方案。Kinect Energy 是北欧的主要能源供应商,依赖于该地区多风气候带来的天然风电资源。
该公司最近推出了一系列 AWS 的 AI/ML 服务。借助 Amazon SageMaker AI,该公司可以预测即将到来的天气趋势,从而预测未来几个月的电力价格,实现前所未有的远程能源交易,这代表了行业领先的前瞻性思维方法。
“我们开始使用 Amazon SageMaker AI,在 AWS ML 解决方案团队和解决方案构架团队的帮助下,我们利用创新日获得了动力,此后其影响一直很大。我们已经多次发展我们的 AI 团队,以充分利用 AWS 技术提供的新优势。我们通过根据尚未发生的天气设定价格来获利。我们已经“全面”使用 AWS,包括将数据存储在 S3 中,使用 Lambda 执行,以及除 SageMaker AI 之外的步骤功能。而且,借助与 AWS ML Solutions Lab 的合作伙伴关系,我们现在可以自给自足,能够迭代构建的模型和持续改进业务。”
Kinect Energy Group 首席业务分析师 Andrew Stypa
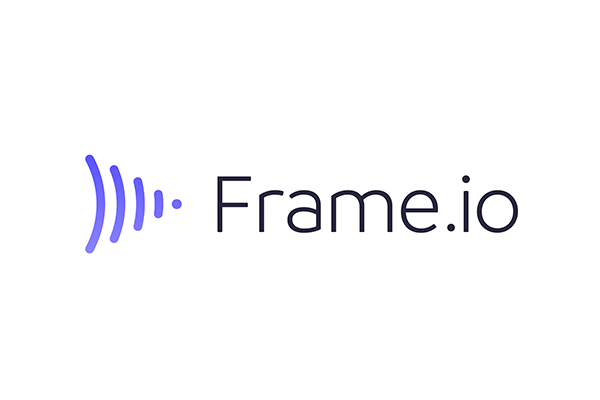
Frame.io
Frame.io 是所有视频的中心。Frame.io 是视频审核与协作领域的领导者,在全球有 70 万客户,从自由职业者到企业等各种视频专业人士都通过 Frame.io 审核、批准和交付视频。
“作为面向全世界用户开放的云原生视频审核与协作平台,为客户提供一流的安全性势在必行。凭借构建到 Amazon SageMaker AI 中的异常检测模型,我们能够利用机器学习快速识别、检测和拦截所有不必要的 IP 请求,以确保我们客户的媒体保持安全并始终得到保护。开始使用 Amazon SageMaker AI、随着时间推移进行维护、跨平台扩展,以及调整以适应具体工作流程一向简单而直接。在 SageMaker AI 中的 Jupyter 笔记本的帮助下,我们能够试验不同模型,从而提高准确度,更快速地找出能够进一步提升 Frame.io 安全性的方法。”
Frame.io 副总裁兼信息安全主管 Abhinav Srivastava
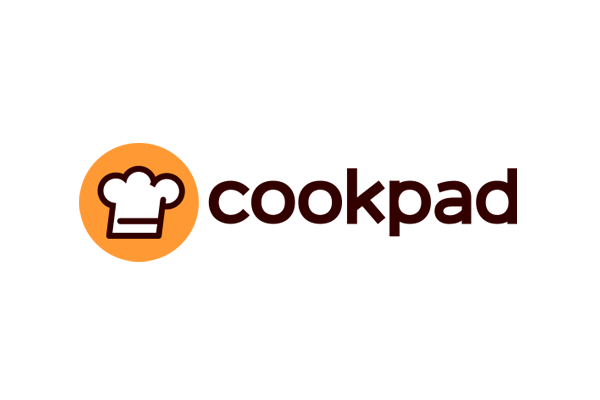
Cookpad
Cookpad 是日本规模最大的食谱分享服务,在日本每月约有 6000 万用户,在全球每月约有 9000 万用户。
“随着对 Cookpad 食谱服务的易用性要求不断提高,我们的数据科学家将构建更多机器学习模型,以优化用户体验。在尝试尽可能减少训练任务的迭代次数以获得最佳性能的过程中,我们认识到部署 ML 推理端点时面临的重大挑战,而该挑战会减慢我们的开发速度。为了自动执行 ML 模型部署以便数据科学家可以自行部署模型,我们使用了 Amazon SageMaker AI 的推理 API,并证明 Amazon SageMaker AI 消除了应用程序工程师部署 ML 模型的需要。我们期望在生产中使用 Amazon SageMaker AI 自动执行此流程。”
Cookpad 研究工程师 Yoichiro Someya
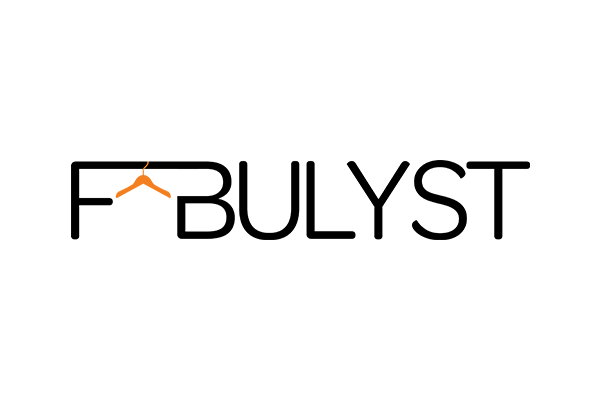
Fabulyst
Fabulyst 是一家专注于时装商贸的印度初创公司,通过人工智能为顾客提供更积极、更个性化的体验,帮助零售商提高转化率。
“Fabulyst 通过将库存商品与用户特定的个性化查询进行匹配(例如适合其身体类型或肤色),让顾客能够更方便地找到最优的商品。此外,我们根据社交媒体、搜索、博客等渠道的数据,使用计算机视觉来预测月度趋势,同时在零售商客户的目录内自动标记这些趋势,从而帮助零售商进一步提高有效转化率。Fabulyst 使用 AWS 来交付先进的解决方案,包括使用 Amazon SageMaker AI 来处理支持我们产品和服务的许多预测。借助 SageMaker AI 和其他 AWS 服务,我们得以向用户保证价值(例如保证零售商的增量收入提升 10%),并且坚信我们始终能够兑现最佳结果。”
Komal Prajapati,Fabulyst 创始人兼首席执行官
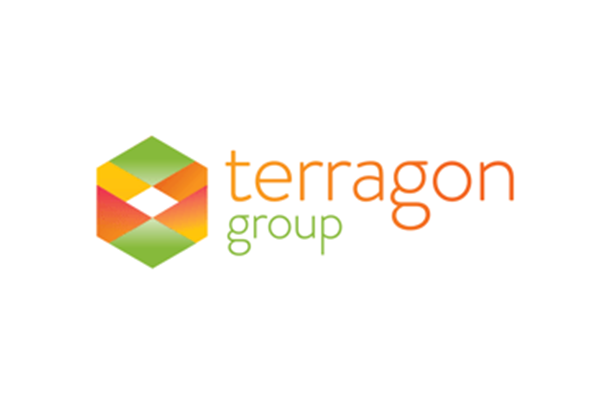
Terragon Group
Terragon Group 是一家数据和营销技术企业,通过洞察信息覆盖非洲的移动用户,为企业挖掘商业价值。多年来,Terragon Group 已经成为移动领域的领导者,服务多个区域的本地和跨国品牌。在合适的时间向合适的用户传递合适的广告信息需要个性化服务,Terragon 使用数据、洞察和人工智能帮助企业在非洲找到正确的受众。
“Amazon SageMaker AI 为我们提供了端到端机器学习工作流程,不需要设置任何底层基础设施。我们的数据科学和机器学习团队能够在短短几个小时内迅速从数据探索进行到模型训练和生产。对于一个位于非洲且工程人才稀缺的企业来说,没有其他方法可以让我们在不到 90 天的时间内建立和部署 ML 模型,以解决现实生活中的问题。”
Terragon Group 首席技术官 Deji Balogun
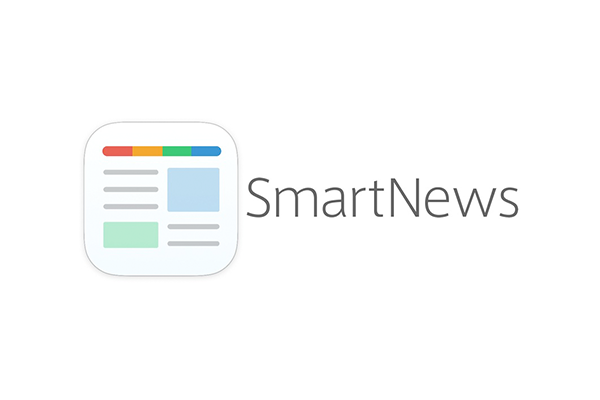
SmartNews
SmartNews 是日本最大的新闻应用程序,每月向全球 1100 多万活跃用户提供优质信息。借助机器学习技术,SmartNews 帮助用户获得最相关、最有趣的新闻故事。SmartNews 的机器学习算法对数以百万计的文章、社交讯号和人类互动进行评估,目前提供了 0.01% 的最重要的新闻故事。
“我们的使命是发现并向世界提供高质量的故事,AWS(尤其是 Amazon SageMaker AI)为我们提供支持,它帮助我们缩短了开发周期,以便更快为客户服务。使用 Amazon SageMaker AI 对我们的新闻策划方法大有帮助,包括使用深度学习进行文章分类,预测生命时间值以及对文本和图像进行复合建模。我们期待通过 AWS 的 Amazon SageMaker AI 和其他人工智能解决方案实现更高的目标。”
SmartNews, Inc. 联合创始人兼首席执行官 Kaisei Hamamoto
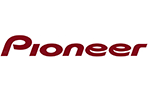
Pioneer
Pioneer 是一家跨国公司,专门从事数字娱乐,包括汽车电子和移动服务。Pioneer 以“触动您的心弦,分享您的感受”为企业理念,为客户提供能够为他们的日常生活带来帮助的产品和服务。
“利用 Amazon SageMaker AI 和 Automatic Model Tuning 等模型训练功能,我们能够开发出高度准确的机器学习模型,并为客户隐私提供持续保护。我们也期待利用适用于机器学习的 AWS Marketplace 获取算法和预先训练的模型,以构建盈利平台。”
Pioneer 信息服务工程部门总经理 Kazuhiro Miyamoto
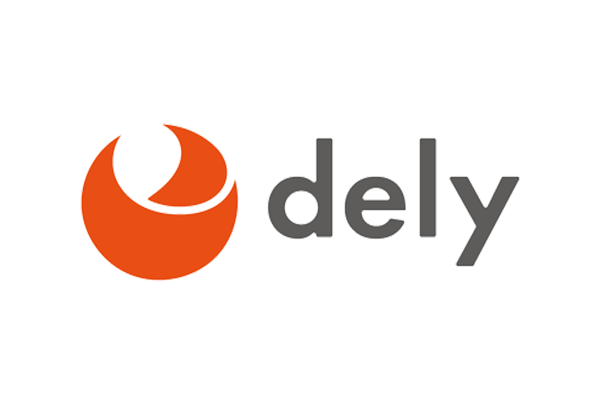
Dely
Dely 运营着日本最好的烹饪视频服务 Kurashiru。让烹饪服务影响世界是它每天努力的目标。Kurashiru 每天都会帮助很多人,它用很多烹饪视频介绍各种美味的食谱,为你的餐桌增添色彩。在日本,有数千万人观看和收听每月的食谱服务。
“自从推出广受欢迎的 Kurashiru 服务以来,我们的移动应用程序在两年半内下载量超过 1500 万次。我们相信,利用机器学习等先进技术,在合适的时间向用户交付合适的内容至关重要。为了实现这一点,我们使用 Amazon SageMaker AI,它帮助我们在 90 天内构建和部署了机器学习模型。我们还通过内容个性化,使点击率提高了 15%。”
Dely, Inc. 首席技术官 Masato Otake
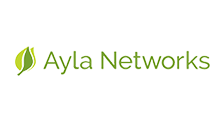
Ayla Networks
Ayla Networks 是一家总部位于旧金山的 IoT 平台即服务软件公司,为消费者和商业市场开发解决方案。
“在 Ayla Networks,我们发现我们的客户主要在 AWS 基础设施上运行,因为它的可扩展性和可靠性得到了验证。特别是,我们发现商业制造商通过 Amazon SageMaker AI 利用 Ayla 云中的设备性能数据。借助 Amazon SageMaker AI 和我们的 Ayla IQ 产品,企业可以获取见解和发现异常,从而提高产品和服务质量,甚至可以预测机器故障并在故障发生之前进行修复。此解决方案可确保我们的客户顺畅运营,以便他们的企业可以毫无顾虑地持续发展、继续生产并扩大规模。”
Ayla Networks 全球营销副总裁 Prashanth Shetty
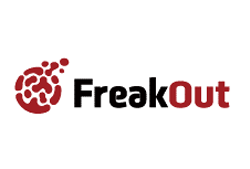
FreakOut
FreakOut 是一家专注于数字广告的领先科技公司。该公司提供用于互联网广告的实时广告库存交易的产品以及网页浏览数据分析。FreakOut 利用机器学习来实现点击率(CTR)和转化率(CVR)预测。
“我们正在将机器学习训练环境从本地迁移到 Amazon SageMaker AI。Amazon SageMaker AI 为我们的业务提供了更具扩展性的解决方案。借助 Amazon SageMaker AI 的 Automatic Model Tuning 功能,我们可以根据自己的要求优化和估计高度准确的模型。”
FreakOut 首席技术官 Jiro Nishiguchi
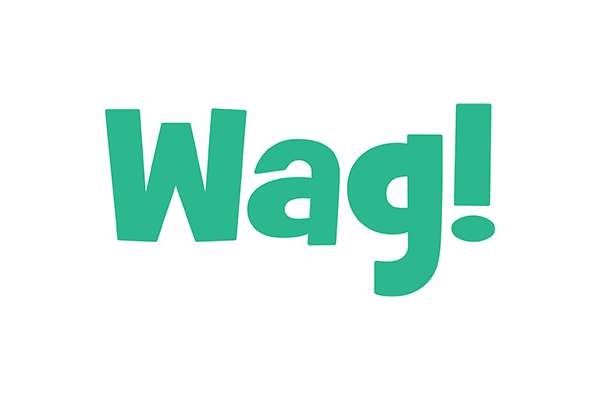
Wag!
“在 Wag!,我们必须满足双向市场中的供需要求。我们发现了使用由 AWS 提供支持的机器学习的机会,可预测客户的遛狗需求。通过在 AWS 上标准化我们的机器学习应用程序,我们能够通过在工程资源受限的情况下以大幅提高的速度和规模进行迭代,满足业务需要的持续增长。利用 Amazon SageMaker AI,我们可以加快机器学习实验,将培训模型所需的 45 天计算时间压缩到 3 天。”
Wag Labs Inc. 工程技术和运营副总裁 Dave Bullock
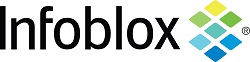
Infoblox
Infoblox 是安全云托管网络服务的领导者,这些服务旨在管理和保护联网核心,即 DNS、DHCP 和 IP 地址管理(统称为 DDI)。
“在 Infoblox,我们使用 Amazon SageMaker AI 构建 DNS 安全分析服务,该服务可以检测恶意创建者,这些创建者通过创建同形异义词来模仿高价值的域名目标,并使用它们来删除恶意软件、通过网络钓鱼获取用户信息和攻击品牌的声誉。AWS 是我们企业的云技术标准,我们可以利用 SageMaker AI 提供的多种功能来加速 ML 模型开发。我们已使用 SageMaker Automatic Model Tuning 功能扩展实验并将准确度提高到 96.9%。得益于 SageMaker AI,作为安全分析服务的一部分,我们的 IDN 同形异义词检测器已识别出超过 6000 万次同形异义词域解析,并且每月继续新发现数百万次解析,这有助于我们的客户更快地检测品牌滥用行为。”
Infoblox 分析架构师 Femi Olumofin

NerdWallet
NerdWallet 是一家位于旧金山的个人理财公司,提供对包括信用卡、银行、投资、贷款和保险在内的金融产品的审核和比较。
“NerdWallet 依赖数据科学和 ML,将客户与个性化理财产品关联在一起。我们选择标准化我们在 AWS 上的 ML 工作负载,因为它使我们能够快速地使我们的数据科学工程实践现代化,从而消除障碍,加快交付时间。使用 Amazon SageMaker AI,我们的数据科学家可以把更多的时间花在战略追求上,把更多的精力放在我们的竞争优势上——我们对为用户解决的问题的洞察力。”
Ryan Kirkman,NerdWallet 的高级工程经理
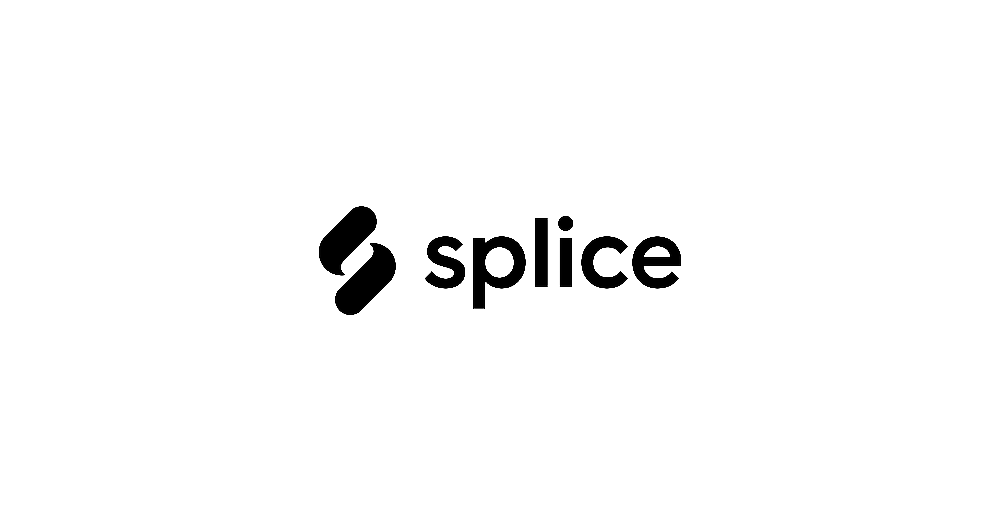
Splice
Splice 是一个音乐家创意平台,由音乐家建立,让艺术家能够释放他们真正的创作潜力。这家订阅制音乐创作初创公司成立于 2013 年,目前服务于 300 多万名音乐人,支持他们在音乐目录中寻找完美的声音。
“随着我们的声音和预设目录不断增加,找到合适声音的挑战也越来越大。这就是 Splice 投资打造一流搜索发现功能的原因所在。通过标准化我们在 AWS 上的 ML 工作负载,我们打造了一个面向用户的全新产品,旨在让音乐家更容易地与他们所寻找的声音联系起来。自从类似的声音推出后,我们发现搜索转化率上升了近 10%。利用 Amazon SageMaker AI,我们为基于文本的搜索创造了完美的补充,从而使我们的用户能够以前所未有的方式发现和浏览目录。”
Alejandro Koretzky,Splice 的机器学习主管兼首席工程师
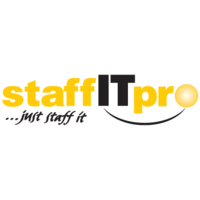
Audeosoft
“在我们开始机器学习之旅之前,我们只能搜索简历 (CV) 的文本,但我们欠缺光学字符识别能力,这意味着并不是所有简历都可以搜索。有了 Amazon Textract,我们现在可以从各种文档提取内容,并且我们有能力在一个 Elasticsearch 集群中为所有上传的文件建立索引。现在,每个上传的文档都可以使用 Elasticsearch 进行搜索,其搜索速度比原来的 SQL 搜索快 10 倍。此外,我们使用 Amazon SageMaker AI 实现了词矢量处理,可以将相关关键词添加到搜索查询中。这个过程使我们能准确地对候选人进行分类和筛选,并帮助我们消除在简历中使用同义词或替代措辞造成的错误。使用 Amazon SageMaker AI 和 Amazon Textract,我们可以为招聘企业提供更聪明、更优质的候选人。性能稳定、全球可用和坚实可靠是 Audeosoft 成功的关键因素。大约 8 年前,当我们决定与 AWS 合作时,我们就知道,未来他们会是优秀的合作伙伴。通过选择 AWS 作为我们首选的云服务提供商,我们拥有了一个在未来几年和我们一样具有创新动力和渴望的合作伙伴。”
Marcel Schmidt, Audeosoft 首席技术官
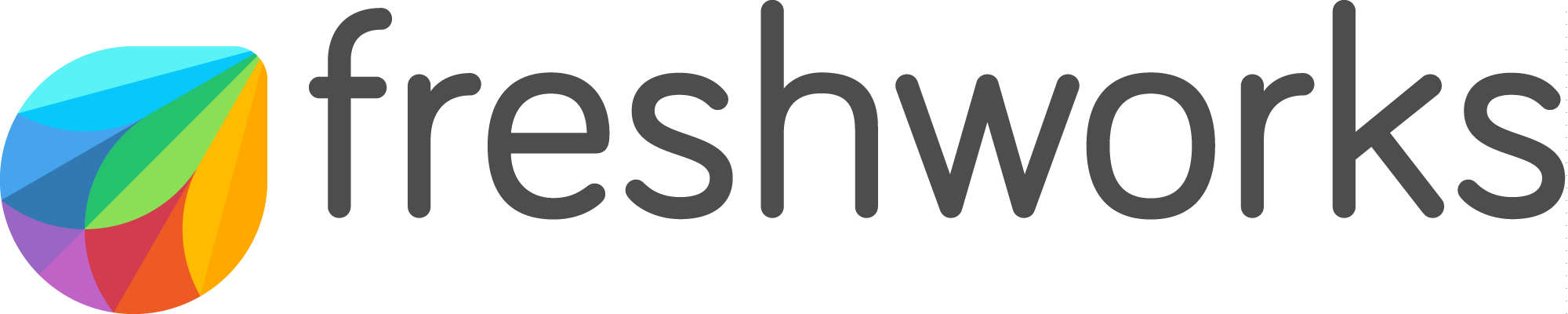
Freshworks
Freshworks 是一家位于美国/印度的 B2B SaaS 独角兽企业,为全球中小企业 (SMB) 和中端市场企业提供服务。Freshworks 为客户和员工参与的工作流程提供了简单易用、功能强大的应用程序产品组合。
“在 Freshworks,我们打造了 AI/ML 旗舰产品 Freddy AI Skills,通过超个性化的模型,帮助代理商解决用户查询并成功解决支持工单问题、帮助销售和市场团队排定机会的优先级别并迅速达成交易,以及帮助客户经理成功降低业务流失风险并促进业务增长。我们选择在 AWS 上标准化 ML 工作负载,因为我们能够轻松构建、训练和部署针对客户使用案例优化的机器学习模型。借助 Amazon SageMaker AI,我们已经为 11000 位客户构建了 30000 余个模型,并将这些模型的训练时间从 24 小时缩短到 33 分钟以下。借助 SageMaker Model Monitor,我们可以跟踪数据偏移并重新训练模型,从而确保准确性。在 Amazon SageMaker AI 的支持下,Freddy AI Skills 在智能操作、深度数据洞察和意图驱动对话等方面不断发展。”
Freshworks Platform 产品高级总监 Tejas Bhandarkar
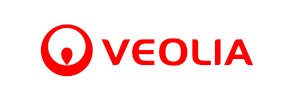
Veolia
Veolia Water Technologies 是一家经验丰富的设计公司,也是水和废水处理技术解决方案和服务的专业提供商。
“在短短八周内,我们与 AWS 合作开发了一个原型,该原型可以预计何时清洁或更换淡化装置中的滤水膜。使用 Amazon SageMaker AI,我们构建了一个 ML 模型,该模型可以从以前的模式中学习,并预测未来污垢指标的演变。通过在 AWS 上标准化我们的机器学习工作负载,我们能够降低成本并防止停机,同时提高所生产的水的质量。这些结果的实现离不开这两个团队的技术经验、相互信任和奉献精神,他们合力实现了这一目标:不间断地供应清洁和安全的水。”
Veolia Water Technologies 首席数字官 Aude GIARD
Sportradar
领先的体育数据提供商 Sportradar 为全球超过 65 项联赛提供实时体育数据。为了提供最前沿的洞察信息,该公司与 Amazon ML Solutions Lab 合作开发了一款足球进球预测器。
“我们特意向 Amazon ML Solutions Lab 团队抛出了一个最棘手的计算机视觉问题,以测试 AWS 机器学习的功能,结果给我留下了深刻的印象。该团队构建了一个 ML 模型,利用 Amazon SageMaker AI 在现场比赛中提前 2 秒预测足球进球情况。仅仅是这个模型就为我们带来了许多新的商业机会。我们期待在 AWS 上标准化我们的机器学习工作负载,因为我们可以构建、训练和部署模型来促进业务创新并满足我们的成本和延迟要求。”
Sportradar 首席技术官 Ben Burdsall
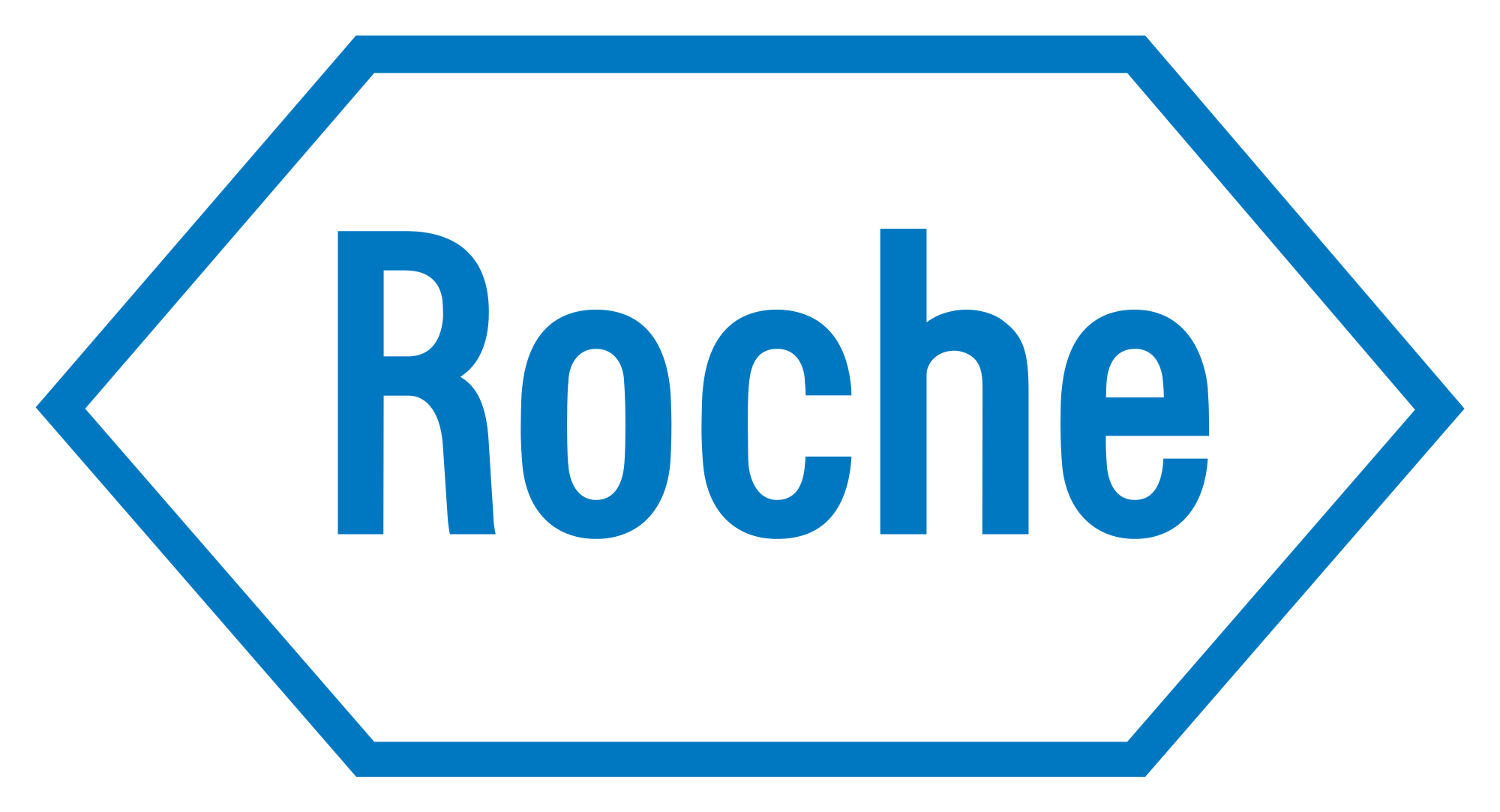
Roche
F.Hoffmann-La Roche AG (Roche) 是瑞士的一家跨国生命科学公司,专门从事制药和诊断。
“我希望推动我的团队在云端系统化我们的 ML 工作流,以便我们可以与 Machine Learning 解决方案实验室合作举办 Amazon SageMaker AI 研讨会,以演示 SageMaker AI 如何为数据科学家简化 ML 生产流程。自举办研讨会以来,我们 80% 的 ML 工作负载在 AWS 上运行,从而帮助我们的团队将 ML 模型的投产速度提高三倍。SageMaker AI 和 AWS 堆栈使我们能够使用计算资源进行按需训练,而不受本地可用性的约束。”
Roche 数据科学家 Gloria Macia
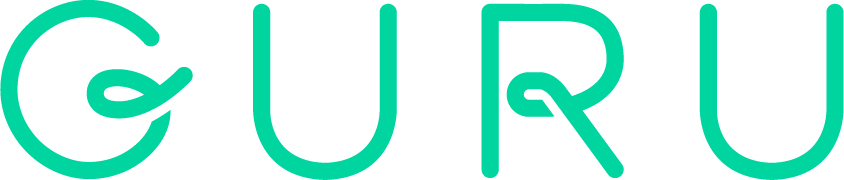
Guru
“在 Guru,我们相信您可以获得执行您的作业所需的知识。我们是知识管理解决方案,可以捕获您的团队最有价值的信息,并将该信息组织到单一事实来源中。我们利用 AI 在您工作的地方实时向您推荐相关知识,确保知识得到验证,并帮助您更好地管理您的整个知识库。我们不断发展的产品数据科学团队面临着现代 ML 团队的所有挑战(构建、训练和大规模部署 ML 系统),我们依靠 Amazon SageMaker AI 来克服这些挑战。目前,我们利用 SageMaker Inference 更快地将 ML 模型部署到生产中,以使它们帮助我们满足首要目标——为客户提供价值。”
Guru ML 高级工程师 Nabin Mulepati
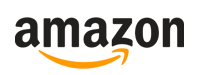
亚马逊运营
作为亚马逊在新冠肺炎(COVID-19)疫情期间对员工安全承诺的一部分,亚马逊运营团队已经部署了 ML 解决方案,以帮助在全球 1000 多个运营大楼中工作的员工保持社交距离。亚马逊运营与 Amazon Machine Learning Solutions Lab 合作,使用 Amazon SageMaker AI 创建用于距离估计的最先进的计算机视觉模型。
“通过在 AWS 上标准化我们的 ML 工作负载并与 ML Solutions Lab 的专家合作,我们创建了一组创新的模型,我们估计可以节省多达 30% 的人力检查工作。借助 Amazon SageMaker AI,我们可以通过每天减少数百小时的人力检查,来将更多的时间集中在安全性和不断提高的准确性方面。”
Amazon OpsTech IT 软件开发总监 Russell Williams
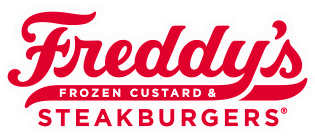
Freddy’s Frozen Custard & Steakburgers
Freddy’s Frozen Custard & Steakburgers 是一家休闲快餐餐厅,可提供独具风味的组合套餐,套餐包含现点现做的牛排汉堡、维也纳牛肉热狗、鞋带薯条和其他带新鲜搅拌冷冻奶油冻的可口小甜食。Freddy's 成立于 2002 年,并于 2004 年获得特许经营权,目前在 32 个州拥有近 400 家餐厅。
“以前,我们只需选择两家看起来相似的餐厅,但是现在我们对菜单项、客户和地点之间的关系有了真正的理解。支持 Domo 的新 ML 功能的 Amazon SageMaker Autopilot 一直是我们营销和采购团队尝试新想法并改善客户体验的助力器。”
Sean Thompson,Freddy's IT 总监
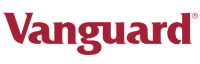
Vanguard
“我们的 Vanguard 数据科学家和数据工程师们现在能够在单个笔记本上进行协作,以执行分析和机器学习,这让我们感到非常兴奋。Amazon SageMaker Studio 拥有与 Spark、Hive 和 Presto 的内置集成,它们都运行在 Amazon EMR 上,因此能够让我们的开发团队更加高效。在这个单一的开发环境中,我们的团队能够专注于构建、训练和部署机器学习模型。”
数据和分析高级总监 Doug Stewart – Vanguard
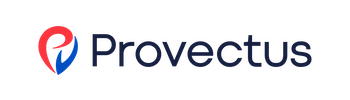
Provectus
“我们一直期待在将来能够直接从 Amazon SageMaker Studio 创建和管理 Amazon EMR 集群,这样,我们的客户就可以直接从 Amazon SageMaker Studio 笔记本运行 Spark、Hive 和 Presto 工作流。Amazon SageMaker AI 现在以原生方式构建了这一功能,以简化 Spark 和机器学习作业的管理,这让我们感到非常兴奋。这样可以帮助我们客户的数据工程师和数据科学家们更有效地协作,以利用基于 EMR 的数据转换来执行交互式数据分析和开发机器学习管道。”
首席执行官 Stepan Pushkarev – Provectus
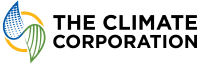
Climate
“在 Climate,我们坚信自己能够为全世界的农民提供准确的信息,帮助他们做出数据驱动型决策,并最大限度地提高他们的每英亩土地回报率。为此,我们投资了机器学习工具等技术,使用称为特征的可测量实体来构建模型,如种植者的田间产量。借助 Amazon SageMaker Feature Store,我们可以通过一个集中的特征存储加快机器学习模型的开发,从而帮助多个团队轻松访问和重复使用特征。通过 SageMaker Feature Store,我们可以针对不同的使用案例,或使用在线存储库轻松地实时访问特征,或使用离线存储库按计划运行特征。有了 SageMaker Feature Store,我们可以更快地开发机器学习模型。”
Atul Kamboj,澳大利亚新南威尔士州政府保险和护理机构 iCare 高级数据科学家;Daniel McCaffrey,Climate 数据和分析副总裁
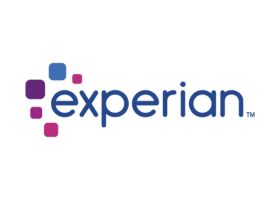
Experian
“在 Experian,我们认为我们有责任让消费者了解和使用理财信贷,并协助贷款人管理信贷风险。随着我们继续实施最佳实践来构建财务模型,我们正在寻找利用机器学习加速产品生产的解决方案。Amazon SageMaker Feature Store 为我们提供了一种安全的方式,用来存储和重复使用我们的机器学习应用程序的特征。能够跨多个账户维持实时应用和批量应用的一致性是我们业务的一项关键要求。利用 Amazon SageMaker Feature Store 的新功能,我们可以帮助客户在新经济中控制信贷并降低成本。”
Geoff Dzhafarov,Experian Consumer Services 的首席企业架构师
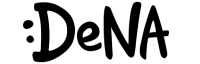
DeNA
“在 DeNA,我们的使命是利用互联网和 AI/ML 带来影响力和令客户满意。提供基于价值的服务是我们的首要目标,我们希望确保我们的业务和服务已准备好实现这一目标。我们希望在整个组织中发现和复用特征,Amazon SageMaker Feature Store 帮助我们以一种简单有效的方式为不同应用复用特征。Amazon SageMaker Feature Store 还帮助我们维护标准特征定义,并帮助我们在训练模型并将其部署到生产环境时采用一致的方法。借助 Amazon SageMaker AI 的这些新功能,我们可以更快地训练和部署机器学习模型,为客户提供最佳服务来让客户满意。”
Kenshin Yamada,DeNA 人工智能系统部总经理
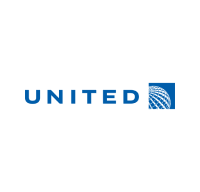
美国联合航空公司
“在 United Airlines,我们使用机器学习(ML)通过提供个性化的服务来改善客户体验,使客户使用 Travel Readiness Center 做好准备。我们对 ML 的应用还扩展到了机场运营、网络规划、航班调度等方面。在我们走出新冠疫情之际,Amazon SageMaker AI 在 Travel Readiness Center 发挥了关键作用,使我们能够使用基于文档的模型自动化处理大量的 COVID 测试证书、疫苗卡。通过 Amazon SageMaker AI 新的治理功能,我们提高了对机器学习模型的控制力和可见性。SageMaker Role Manager 通过为与 IAM 角色相关的每个角色提供基线权限和 ML 活动,极大地简化了用户设置过程。通过 SageMaker Model Cards,我们的团队可以主动捕捉和分享模型信息,以供审查,而使用 SageMaker Model Dashboard,我们能够搜索和查看部署在 MARS(我们内部 ML 平台)上的模型。凭借这些新的治理功能,我们节省了大量的时间,并且得以纵向扩展。”
United Airlines ML 工程和运营总监 Ashok Srinivas
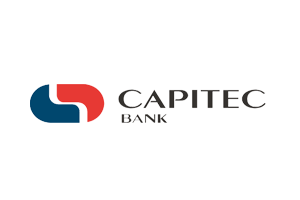
Capitec
“在 Capitec,我们产品线上的数据科学家们各有所长,可以构建不同的 ML 解决方案。我们的 ML 工程师们管理着一个建立在 Amazon SageMaker AI 上的集中式建模平台,以支持所有这些 ML 解决方案的开发和部署。由于没有任何内置的工具,跟踪建模工作往往会出现文档不连贯和模型不可见的情况。通过 SageMaker Model Cards,我们可以在一个统一的环境中跟踪大量的模型元数据,而 SageMaker Model Dashboard 为我们提供了每个模型性能的可见性。此外,SageMaker Role Manager 简化了我们不同产品线中数据科学家的访问管理过程。这些都有助于我们凭借治理模式,保证客户对我们作为金融服务提供商的信任。”
Capitec 银行 ML 工程师 Dean Matter
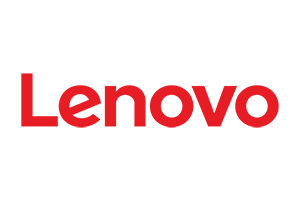
联想
全球第一大 PC 制造商 Lenovo™ 最近将 Amazon SageMaker AI 纳入其最新的预测性维护产品中。 Ashok Srinivas,United Airlines ML 工程和运营总监。
“新的 SageMaker Edge Manager 将帮助消除部署后优化、监控和持续改进模型所需的手动工作。在它的帮助下,我们预计我们的模型将比其他类似机器学习平台运行得更快、消耗的内存更少。SageMaker Edge Manager 允许我们在边缘自动对数据进行采样,将其安全地发送到云中,并在部署后持续监控每个设备上每个模型的质量。这使我们能够远程在全球的边缘设备上监控、改进和更新模型,同时节省我们与客户的时间与成本。”
Lenovo PC 和智能设备云与软件副总裁 Igor Bergman

Basler AG
Basler AG 是为工业、医药、运输和其他各种市场提供高质量数码相机和配件的领先制造商。
“Basler AG 在众多行业中提供智能计算机视觉解决方案,包括制造、医疗和零售应用。我们很高兴通过 Amazon SageMaker Edge Manager 带来的新功能来扩展我们的软件产品。为了确保我们的机器学习解决方案具有可靠的性能,我们需要一个可扩展的边缘到云机器学习运营工具来帮助我们持续监控、维护和改进边缘设备上的机器学习模型。SageMaker Edge Manager 允许我们在边缘自动对数据进行采样,将其安全地发送到云中,并在部署后持续监控每个设备上每个模型的质量。这使我们能够远程在全球的边缘设备上监控、改进和更新模型,同时节省我们与客户的时间与成本。”
Basler 软件解决方案主管 Mark Hebbel
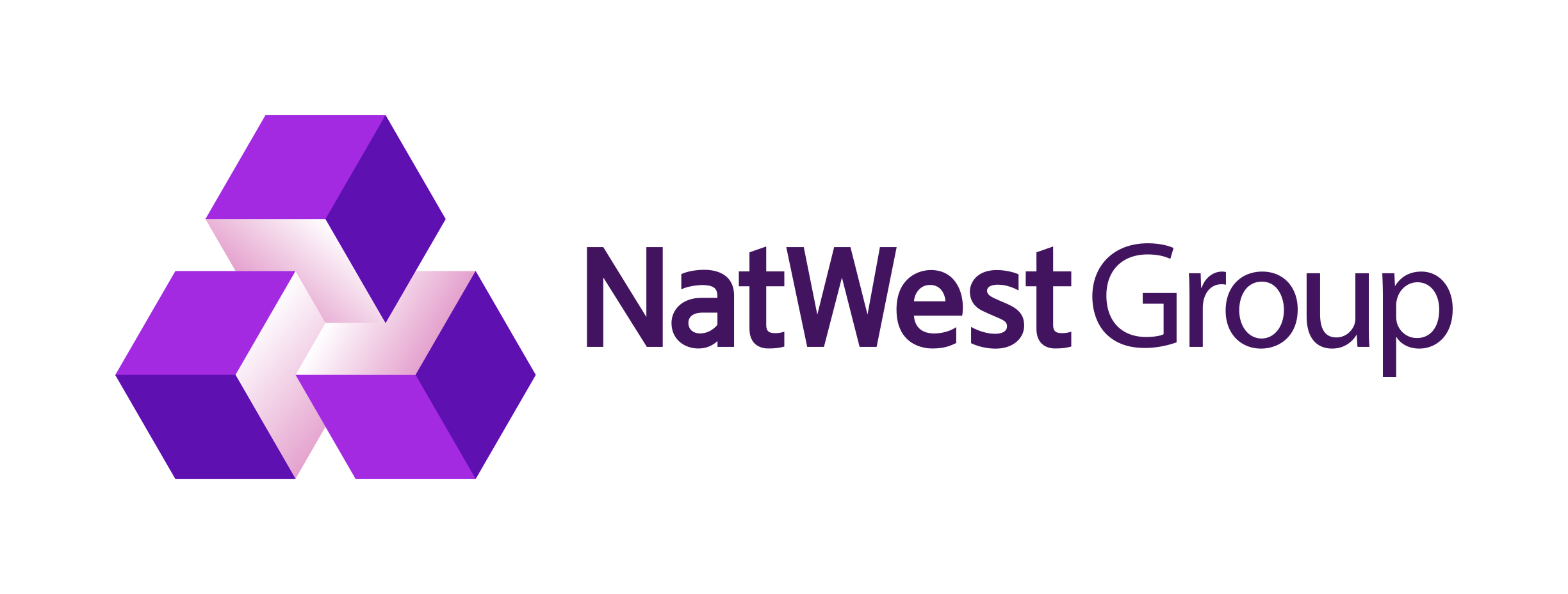
NatWest Group
NatWest Group 是一家大型金融服务机构,实现了组织内机器学习模型开发和部署流程的自动化,将创建新机器学习环境的周转周期从 40 天缩短至 2 天,并将机器学习用例实现价值的时间从 40 周加速到 16 周。
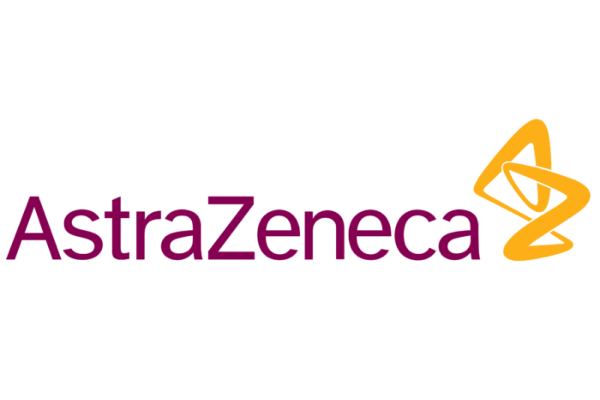
AstraZeneca
“我们可以在 Amazon SageMaker Studio 中轻松自动化大部分机器学习开发流程,而不需要创建许多手动流程。”
Cherry Cabading,AstraZeneca 全球高级企业架构师
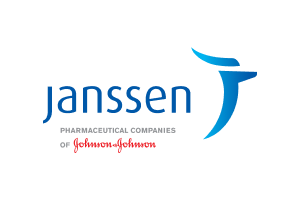
Janssen
利用 AWS 服务(包括 Amazon SageMaker AI),Janssen 实现了自动化 MLOps 流程,将模型预测的准确度提高了 21%,特征工程的速度增加了约 700%,在降低成本的同时,提高了效率。
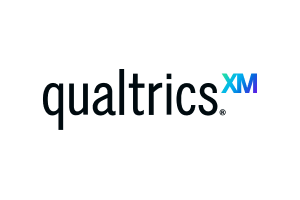
Qualtrics
“Amazon SageMaker AI 使用大规模测试和部署机器学习模型所需的工具,提升了我们 MLOps 团队的效率。”
Qualtrics 机器学习工程师 – Samir Joshi
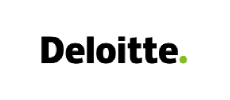
Deloitte
“Amazon SageMaker Data Wrangler 使我们能够利用丰富的转换工具集来满足数据准备需求,这些转换工具可加快将新产品推向市场所需的机器学习数据准备过程。反过来,我们的客户可以从我们扩展部署模型的速度中受益,这使我们能够在几天而不是几个月的时间内提供可衡量、可持续的结果,以满足客户的需求。”
Frank Farrall,Deloitte AI 生态系统和平台首席负责人
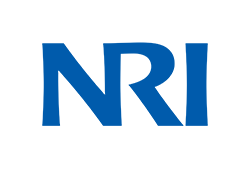
NRI
“作为 AWS 高级咨询合作伙伴,我们的工程团队正在与 AWS 紧密合作,以构建创新的解决方案来帮助我们的客户不断提高其运营效率。机器学习是我们创新解决方案的核心,但是我们的数据准备工作流程涉及复杂的数据准备技术,因此在生产环境中投入使用需要花费大量的时间。借助 Amazon SageMaker Data Wrangler,我们的数据科学家可以完成数据准备工作流程的各个步骤,包括数据选择、清理、探索和可视化,这有助于我们加速数据准备过程并轻松准备用于机器学习的数据。有了 Amazon SageMaker Data Wrangler,我们可以更快地为机器学习准备数据。”
Shigekazu Ohmoto,NRI Japan 高级企业常务董事
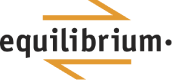
Equilibrium
“随着我们在人口健康管理市场中持续扩展到更多健康支付机构、提供商、药品福利管理者和其他卫生机构,我们需要一种自动完成端到端流程的解决方案,作为向机器学习模型提供信息的数据来源,包括索赔数据、参加数据和药店数据。使用 Amazon SageMaker Data Wrangler,我们现在能够使用一组更容易验证和重复利用的工作流程缩短为机器学习聚合和准备数据所用的时间。这极大地提高了我们模型的交付速度和质量,提升了数据科学家的效率,并将数据准备时间缩短了接近 50%。此外,SageMaker Data Wrangler 还帮助我们节省了多重机器学习迭代并显著减少了 GPU 时间,为客户加快了整个端到端流程的速度,因为我们现在能够构建具有数千种特点的数据集市,包括药店、诊断代码、ER 访视、住院以及人口统计学和其他社会决定因素。通过 SageMaker Data Wrangler,我们可以极其高效地转换数据以构建训练数据集,在运行机器学习模型之前根据数据集生成数据见解,并为大规模推理/预测准备真实数据。”
Lucas Merrow,Equilibrium Point IoT 首席执行官
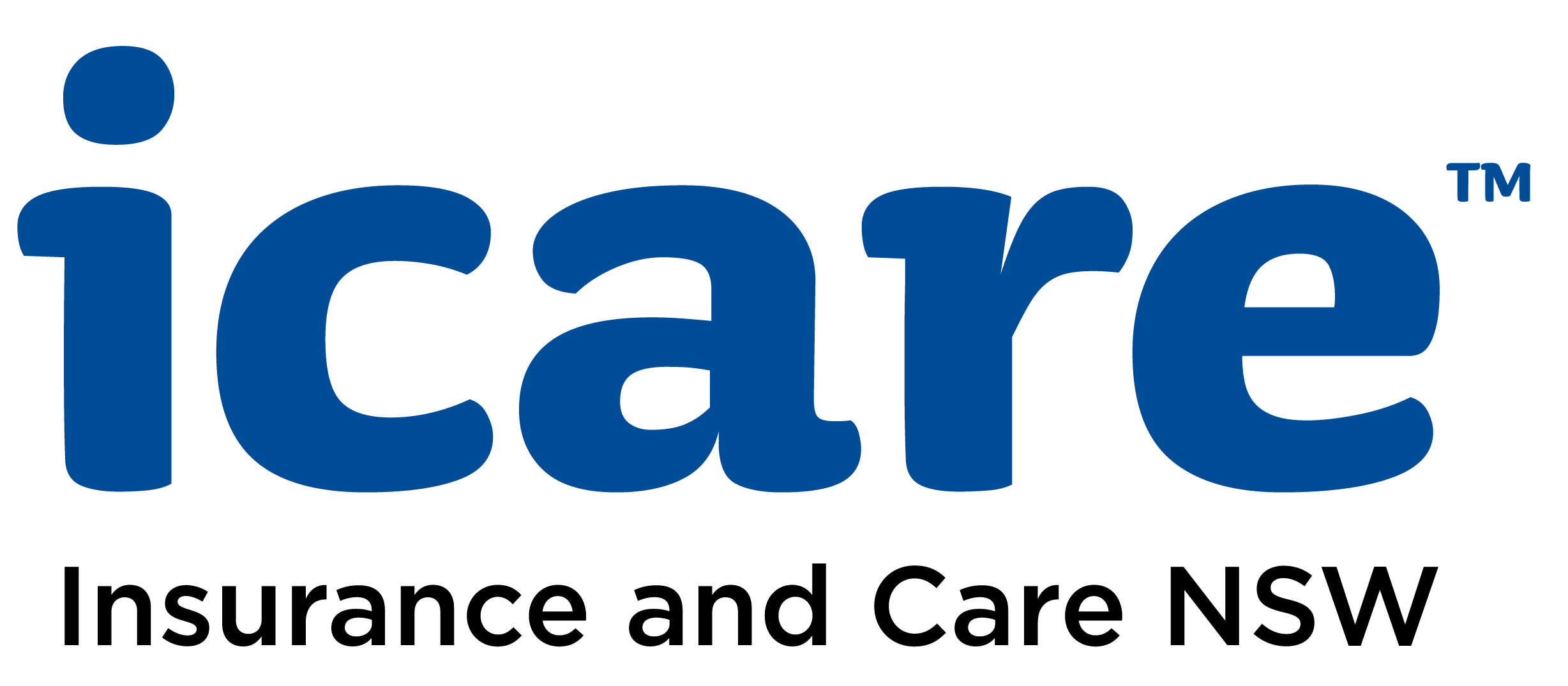
icare Insurance and Care NSW
iCare 是新南威尔士州一家政府机构,为澳大利亚新南威尔士州 329,000 多名公共和私营部门雇主及其 320 万名员工提供工伤补偿保险。此外,iCare 还为建筑商和房主提供保险,为在新南威尔士州道路上受重伤的人提供治疗和护理;并保护超过 2666 亿加元的新南威尔士州政府资产,包括悉尼歌剧院、悉尼海港大桥、学校和医院。
“在新南威尔士州保险与护理公司(iCare),我们的愿景是改变人们对保险和护理的看法。Amazon SageMaker AI 使 iCare 能够构建和训练深度学习模型,以便及早识别长期粉尘病患者。这种早期识别可以防止出现危及生命的情况。根据之前的研究,在 39% 的患者中,矽肺病的迹象被遗漏或无法检测到。人工智能辅助诊断使医生能够正确识别出 80% 的病例,而非辅助诊断的这一比例为 71%。实施该项目后,我们将为 Amazon SageMaker AI 提供资源,以在其他项目中开发解决方案和流程,事实证明,这比以前更快、更容易,而且我们能够轻松扩展我们的工作,为新南威尔士州的人民提供医疗服务。”
Atul Kamboj,澳大利亚新南威尔士州政府保险和护理机构 iCare 高级数据科学家