
The Seattle Seahawks employ AWS powered data-driven tools to shape their success on and off the field.

![There are thousands of draft eligible players but a scout can only watch so many players,” Ward explains. “But [with AWS], we can run models against all players and identify unique guys that stand out or bring people into the fold that haven’t gotten the same amount of evaluation as other players—because they played at a smaller school or played in a smaller program. There are thousands of draft eligible players but a scout can only watch so many players,” Ward explains. “But [with AWS], we can run models against all players and identify unique guys that stand out or bring people into the fold that haven’t gotten the same amount of evaluation as other players—because they played at a smaller school or played in a smaller program.”](https://d1.awsstatic.com/042723_Seahawks_data.f1568be6a6ca3e31b9fec4e0eb5bca256b2041ec.jpg)
“There are thousands of draft eligible players but a scout can only watch so many players,” Ward explains. “But [with AWS], we can run models against all players and identify unique guys that stand out or bring people into the fold that haven’t gotten the same amount of evaluation as other players—because they played at a smaller school or played in a smaller program.”
Patrick Ward - Seattle Seahawks’ head of research and analytics
-
What's one of the secrets to building a successful draft class?
We sat down with Seattle Seahawks’ head of research and analytics, Patrick Ward, for a behind-the-scenes look at how AWS harnesses the collective expertise of coaches, scouts, and analytics to acquire knowledge about players heading into the NFL Draft.
MAKING THE SWITCH FROM ANALOG TO DIGITAL
Data analysis has come a long way since Ward began his career in the football industry over a decade ago. When he first started, the collection process was entirely manual. After games and practices, he and his team would work tirelessly through the night to build reports gathered from multiple technology systems and sources—sifting through things like personal laptops, spreadsheets, text files and multiple databases. It was a lot of legwork.
“Every night we would run a report, join these datasets, go to these folders, grab these datasets, fit this model, run this model. Everything was dependent on the person who was running things.” Ward admits that without a reliable system and way to share queries with staff in other departments, he and his team often risked the chance of delivering disconnected data and siloed analytic reports.
But the NFL is always evolving and the league started shifting toward adopting more analytical approaches to understanding the game. And as a data-driven organization, the Seahawks were looking to gain a competitive edge by gathering insights faster from an explosive growth in data volumes and types.
By leveraging AWS technologies, the Seahawks have been able to shift their operational approach. They’ve successfully consolidated their data collection, storage, and catalog into one single data lake—with personalized web applications that help pin-point unique player perspectives, at the touch of a button. Now, with everything in one place, they are able to easily streamline and share information across their organization, without staff members having to rely on an analyst to build out new reports. For Patrick and team, this was a challenge that was worth solving.
“Data is only increasing. So, putting systems in place to handle this data is critical to stay on the cutting edge of player analytics.”
EARNING A SEAT AT THE DRAFT TABLE
Since moving over to AWS, the Seahawks’ drafting formula has evolved. Take a peek in the Seahawks draft room and you’ll notice Ward and his team have a seat at the table—a far cry from the once exclusive “scouts only” rule. During draft season, you’ll find analysts front and center at every meeting. Leveraging and interpreting personalized data collected by AWS custom web apps that help identify prospects who may have otherwise fallen through the cracks.
“There are thousands of draft eligible players but a scout can only watch so many players,” Ward explains. “But [with AWS], we can run models against all players and identify unique guys that stand out or bring people into the fold that haven’t gotten the same amount of evaluation as other players—because they played at a smaller school or played in a smaller program.”
Between the draft selections, undrafted free agents, and rookie minicamp, the Seahawks end up bringing in roughly 25 prospects each offseason. And similar to players like Doug Baldwin or Brian Mone, there are a lot of athletes in the back end of the draft and into free agency that have an opportunity to get on the field, make their mark, and have a positive career. But for whatever reason, they fall through the cracks and you're just hoping that you can identify them and see if there's an opportunity for them to contribute.
“A lot of it for us is being able to construct systems which can aggregate data across all potential draftees and then build and leverage models to help compare talent, organize talent [and create] short rankings for players—ultimately [we’re] trying to forecast what we think this player may become in the NFL.”
USING AWS TO BUILD A FUTURE PROOF INFRASTRUCTURE
After experiencing the benefits through a collaborative work stream, the Seahawks introduced these systems in other ways throughout the organization. Today, they are utilizing data lakes to gain a deeper understanding of player capabilities. Being able to use the data lake cross-functionally has helped him and his team maximize the value of data, giving the Seahawks a competitive edge on and off the field.
So, what kinds of information is his team leveraging to predict success? Applied physiology, for one. Custom built dashboards geared toward the draft easily organize metrics that better help Patrick evaluate things like health, risk tolerance and the best ways to practice a player. The data is then shared directly with the appropriate decision-makers where it is evaluated and analyzed.
The Seahawks have built a pretty considerable database around the structure and contents of practices that allows them to build unique models specific to their coaching staff, players, and the natural events throughout a given season. Using AWS technologies, they are able to ingest a practice script, run a model against it, and, as a result, give the staff a report on estimated training demands given what we know about that day’s events. This script includes parameters coaches have specified in drills, even down to the individual player level and potential risks to manage. This new level of insights has allowed them to have unique discussions about the contents of practice that were never previously not possible.
“We’re getting more complex and diverse types of data. We need a system that allows us to create an infrastructure that is future proof. [AWS technologies] allows us to have a data lake that can ingest everything from simple count data to tracking data to video data. We are able to build purpose-built layers around that that allows analysis to happen quickly.”And when it comes to data analysis, speed is key. The Seahawks’ centralized data lake has led Patrick and his team to insights that can be used to help solve a variety of problems. And with information in one place they are now able to quickly construct individualized web apps that can answer key questions and meet the needs of each and every coach.
“Being agile enough to meet [the coaches] needs while building something more, that is future proof. And being able to provide other coaches with similar information is critical."
LEANING INTO DECISION MAKING DATA
When it comes to football, data is power. And it’s evolving, fast—with more and more complex data sets being aggregated daily. The benefit? Time and agility. “[AWS technology] quickly gets us from ‘What are we going to do with all of this data?’ to directly putting it in the hands of the end user, the decision makers, and the people who need to interact with it,” Ward says. “Edges in sport are fleeting—as soon as you feel like you’ve got an edge, 60 days later everybody’s got that same edge. You’ve got to move fast and iterate quickly.
”So, what exactly IS the secret to a successful draft? Well, if you ask Patrick you might just get your answer: “Everybody is looking at the same types of information. Everybody’s trying to ask the same questions. The unique part is the ways in which we use AWS to reveal deeper insights and bring all of that information together.”
-
Under the Hood: How the Seahawks Use AWS to Apply Data-Driven Insights Across the Franchise.
An exclusive article written by the Seattle Seahawks that details how AWS technology represents a path forward for their football operations department. It includes details how an AWS data lake helps them build, store, and analyze information across player acquisition, team and opponent scouting, and player health and performance.
Introduction
The National Football League (NFL) is the highest level of competitive football in the world. Each year, 32 teams compete in a 17-game regular season, attempting to qualify for the playoffs and make a run at being crowned Super Bowl champion. Unlike the sport of baseball, a paucity of research exits around player performance and their corresponding contribution on the field. Such a void is centered around the interaction of players on each play, which ultimately determines whether the offense or defense will win the down. While simple count statistics (yards gained, touchdowns, tackles, etc.) have been traditionally collected and reported, newer data streams, largely driven by advances in technology, have provided teams with the ability to begin to untangle such relationships between players and better understand performance.
At the Seattle Seahawks, we create methods for acquiring and storing data and then help to direct the research and analysis efforts within football operations. These efforts are broadly defined within three domains: (1) Player health and performance; (2) Player acquisition; and (3) Team and opponent scouting. Across these three domains our research and analytics department’s primary objective is to acquire, store, and clean data and then conduct relevant analysis specific to the needs of the decision-makers within those departments. As data has grown from the simple count statistics into large sets of player tracking data across NCAA and NFL competitions there is a need now, more than ever, to ensure an infrastructure is in place to help the team derive meaningful insights from this information. Our partnership with the engineers at AWS has been front at center at taking our data pipeline to the next level, allowing for a faster sharing of information across football operations.
Data Utilization Within Football
As technology and data providers have increased so has the evolution surrounding information about how the game is played. Currently, we ingest data relating to players in the NFL and NCAA, which includes not only basic stats, but advanced stats provided by various companies that manually code events within the game, and large amounts of player tracking data. In addition, in house data collection pertaining to player health, such as inertial sensor data worn during practice and force plate data collected during jumping activities, is also pertinent to the weekly workflow of our football team.
While teams have traditionally functioned by storing data in dispersed data stores and spreadsheets, performing analysis as questions arise, such a strategy is no longer adequate given the volume of data we now have access to. Utilizing the AWS best practices for ETL and data management to retrieve the data and then organize and store it within our Redshift database has allowed us to provide rapid insights to those in football operations. Such speed is made possible by leveraging tools including AWS S3, AWS Step Functions, AWS Lambda Functions, AWS Fargate, AWS EventBridge, AWS DMS, AWS DynamoDB, and AWS Glue Jobs to ingest the raw data and create aggregated tables that combine the raw data in meaningful ways for analysts and developers. We use AWS Step Functions as the core serverless automation piece performing the ETL that pulls data from our many data sources into S3. Within those Step Functions we leverage Lambda Functions, DMS, and Fargate to perform different functions depending on the work required to perform the necessary extract, transform, and load processes. Each of these tools get scaled as needed for their specific tasks. Settings and configuration information are stored in DynamoDB with scheduling information in EventBridge. Glue jobs are used to move data from S3 into Redshift.
Following data ingestion and aggregation, analysis is performed, and the result is shared within our in-house application and Shiny web applications. This allows the relevant users to visualize data in informative ways and drive additional questions. The following example is motivated by such a workflow.
The combination of inertial sensors, in-game radio frequency data generated by player worn sensors (NextGen), and jump data captured weekly via force plate are used to inform the team’s performance staff about the individual player’s readiness to tolerate the upcoming week of preparation leading into the next game. With a game being played every seventh day, ensuring that players recover optimally and “win the week” with respect to physical, mental, and tactical preparation is imperative to high levels of success on game day. Previously, without AWS, we would store such information across multiple CSV files and join them together to produce daily and weekly reports. Such an approach is not sustainable and requires a substantial amount of heavy lifting on the part of the analyst to always be present to run these reports and ensure the data is properly stored and coded properly within the CSV sheets. Moreover, these reports were static, meaning that performance staff members must parse through them to find information that is relevant to a player or problem they are attempting to solve for.
Using AWS, the data is ingested automatically via API to our RedShift database using Step Functions and the associated tools shown in Figure 1. Queries are then run against these data sources and a data set is produced that can be analyzed for daily and weekly insights regarding player health and well-being. The analysis is run within the AWS cloud, generating a table of outputs for the performance staff. These outputs are then shared across the department using a Shiny web app, offering a user interface that allows performance staff members to query they data in various ways (e.g., by position, by player, by date range, by training day or training week), depending on the question they are attempting to answer. This analysis is discussed by the staff and used to help plan training for the week based on individual player’s needs. Once players are identified to be outside of a normal range or on a potentially unwanted trend, requiring an intervention to mitigate any unintended consequences to player health or performance, additional analysis is conducted, which ingests the training plan for the upcoming week, providing an expected workload on each of the upcoming days conditional on how the training plan has been constructed (Figure 2). Collectively, this information is then used to assist the Performance Director in developing a bespoke training plan for each athlete leading into the next game.
Conclusion
The development of our AWS technology infrastructure represents a path forward for our football operations department. The construction of our data lake has allowed us to interact with diverse data sets and construct data sets for asking unique football questions which help the team in prepare for upcoming opponents, evaluate player performance, and manage player health. Integrating our AWS infrastructure with other AWS partners, such as Posit Shiny web apps, allows us to leverage model building and output sharing with decision-makers across the organization.
"Data is only increasing. So, putting systems in place to handle this data is critical to stay on the cutting edge of player analytics.”
Patrick Ward - Seattle Seahawks’ head of research and analytics
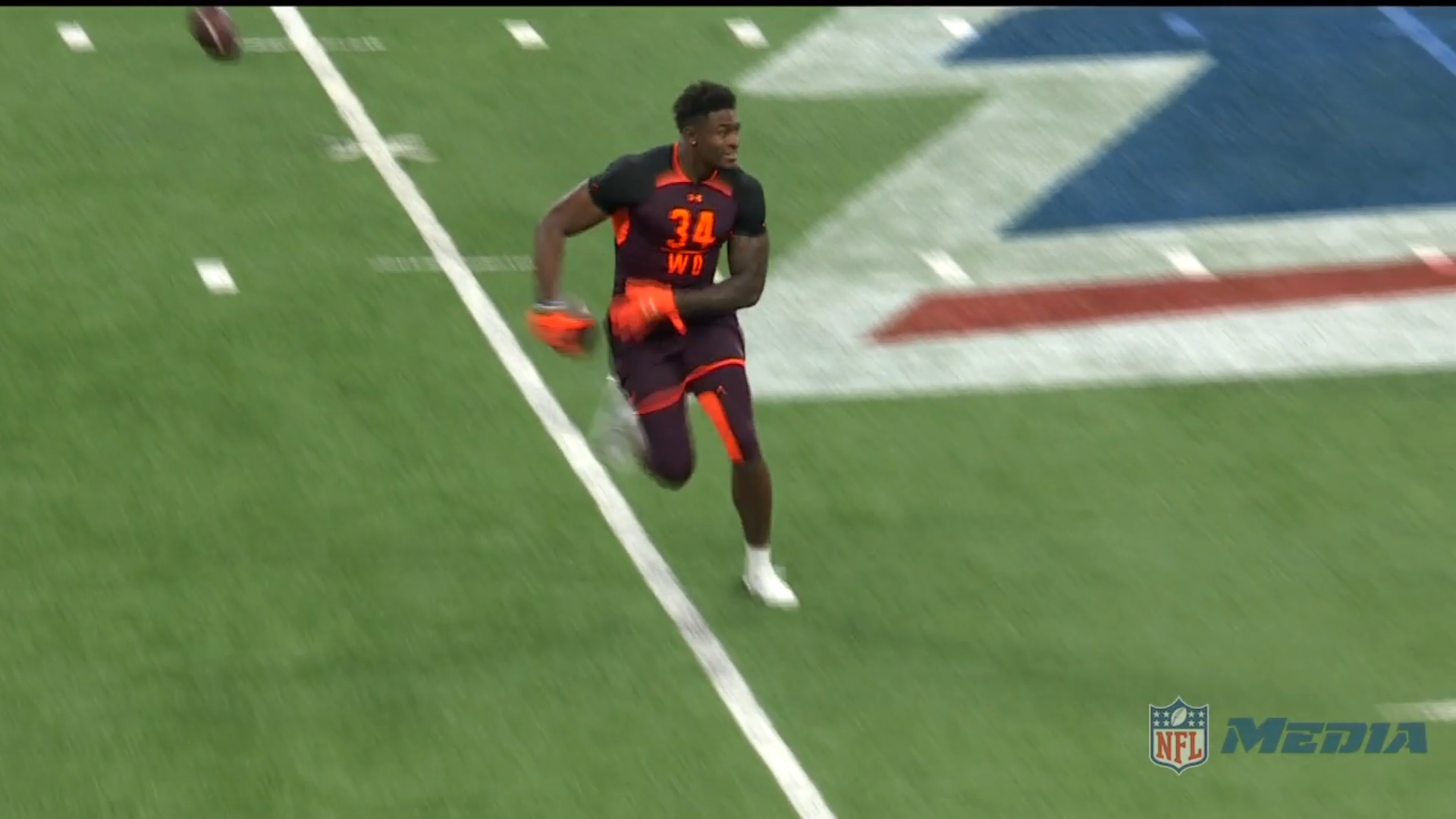
Ready to learn more about how AWS can help accelerate your business?
