AWS Startups Blog
With Nyansa, Big Data Network Analytics Meets the Cloud
Guest post by David Callisch , VP of Marketing at Nyansa
Today’s modern corporate access network is under siege.
As smart device-armed mobile users continue to multiply and access more and more media-rich cloud-based applications, IT staffs have become overwhelmed with the massive volumes of disparate data that must be analyzed and correlated to find and fix problems impacting users’ network experiences.
Conventional infrastructure management solutions, while useful, were simply never developed for this modern day and mobile age, nor designed to deal with the analysis and correlation of so much data across the entire network.
These traditional tools look at performance from a network perspective without the context needed to address client performance problems hiding in the network that negatively impact user productivity.
Recent advances in big data analytics, cloud computing, and machine learning technologies, however, are now being used to solve these problems–driving the emergence of new, cloud-based big data network analytics SaaS solutions.
These solutions have been developed to help companies quantify and manage user performance across corporate IT infrastructure using real data from real users. Something not possible until now.
The confluence of these technologies is why companies can now transform network operations from a cost to a profit center, gain visibility into network blind spots, and use existing data running over their networks to improve top line revenue.
SO WHAT’S NEEDED?
To gain maximum visibility into the user network experience, IT operations staff need insight into the client device’s ability to associate in a timely manner to a wireless access point, authenticate via RADIUS, obtain an IP address via DHCP, resolve domain names via DNS and finally transmit/receive data from the internet.
A hiccup with any individual step can result in service disruption and poor performance, but the user just chalks it up as a problem with “the network” (and probably as “a problem with IT”).
But for IT, was it a device OS problem? A Wi-Fi issue? DHCP? ARP? DNS? An application failure? A WAN problem? More importantly, how can you find the answer without having to scour through volumes of log data, packet captures, and pretty screens and graphs generated by various vendor management systems?
Maintaining complete awareness of all of these user network transactions is a daunting duty for network managers who must sift through troves of network, application, and user data from a variety of different domain silos. It’s virtually impossible to any network professional to have an intimate knowledge of every flavor of operating system running on every smart mobile device, or how these devices interact with all the different parts of the network. Understanding these interactions must be automated for organizations to get a good grip on actual user performance on corporate networks. Enter big data network analytics as a service.
A CLOUDY FUTURE FOR INFRASTRUCTURE MANAGEMENT
New network analytics platforms, acting as a public or private SaaS solution are redefining traditional enterprise infrastructure management.
These systems collect wired packets, device data, wireless metrics, applications and WAN data. These systems crunch all ingested data sources concurrently to understand important patterns and trends impacting user network performance from virtually any vantage point.
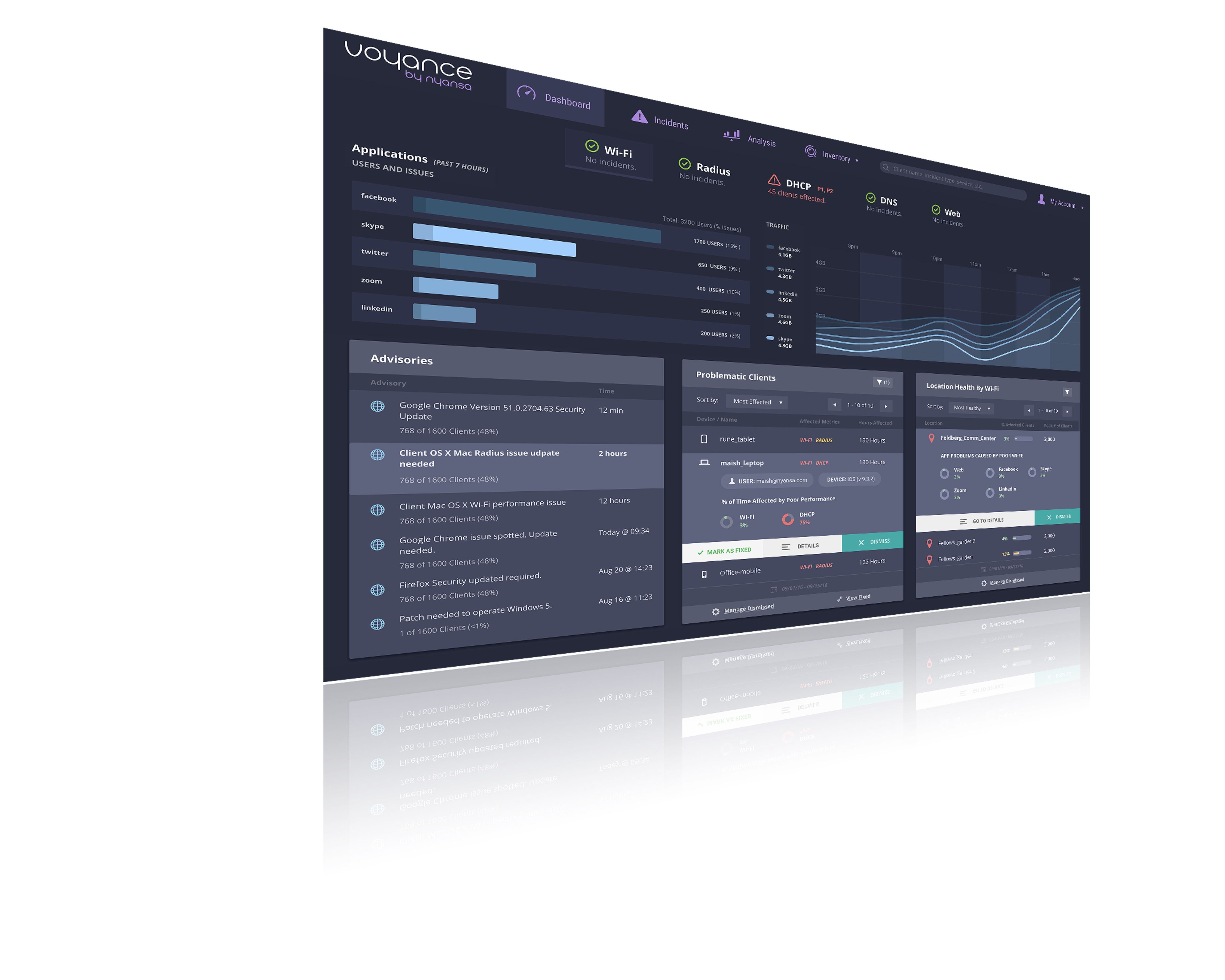
Figure 1: Voyance by Nyansa Analytics Dashboard
Voyance by Nyansa is one such example of the evolution of infrastructure management. Voyance leverages the power of cloud-based Web services like AWS, time-series data bases (i.e. Casandra), big data analysis engines (e.g. Apache Spark) and sophisticated search technologies (e.g. Elastic Search) , among other things, to create a highly scalable analytics engine that can analyze and correlate petabytes of data– turning it all into actionable insights that would take days or months of manual data crunching.
These new analytics-based solutions constantly stare at all parts of the network, watching and learning the behavior of client transactions up and down the stack as they happen, storing this data historically to identify larger patterns and trends that emerge.
Network traffic is typically spanned from a mirrored network port to a software collector that sits out of band. This software collector also gathers other vital data from network elements like wireless LAN controllers, routers, UC systems, AAA, DHCP and DNS servers as well as client and IoT devices using SNMP, APIs and other means.
This then creates a single source of network truth across the entire network that can then be used by all IT factions who need to understand what’s really happening, when, where and why.
Once the data is ingested, it is analyzed, compared, compressed and securely send to a backend analytics engine that presents the data in plain English for IT staff to use. No packets or sensitive user data is ever exposed.
These new analytics platforms are uniquely positioned to help IT be more proactive by learning how different parts of the network are working (or aren’t working) together. They then suggest, a la Netflix, what to do about it, giving network managers a list of configuration recommendations to fix specific client incidents or systemic infrastructure problems impacting user performance across the entire infrastructure.
This new class of solution is focused on this approach has been coined “user performance management” (UPM). Although still in their infancy, UPM platforms promise to fundamentally alter the traditional reactive workflows of IT.
CONCLUSION
As access network architectures evolve, it will become increasingly difficult to associate user performance with any single application given so many network interdependencies that make optimal user performance possible.
Big data network analytics SaaS solutions now provide an easy and affordable way to track how these devices are performing by constantly analyzing every communication across all layers of the IP stack. Until now, this hasn’t been operationally feasible.